Within the data science world, a number of young companies are on the rise. Databricks is an example of this. By making open-source leadingfor its software, the company has gained a foothold in a relatively short period of time. We recently visited the company’s Spark + AI Summit, where we spoke with Principal Software Engineer Michael Armbrust.
Databricks’ trick is to develop frameworks and then make them open source. Usually, the software is donated to the Apache Software Foundation, but recently the Linux Foundation has also become a destination. Without a doubt, the best-known solution – which now falls under a foundation – is Apache Spark. Many developers are already working with and on this software.
Databricks also uses the frameworks themselves for its Unified Data Analytics Platform. This is an excellent platform for employees specialised in machine learning and data. Think of data scientists, data engineers and data analysts. The platform provides them with tools to tackle challenges related to their work. The philosophy of Databricks is to extract real value from data, by means of those tools.
Problems according to Databricks
Given the complexity involved in converting data into valuable insights, retrieving valuable data can be quite difficult. Anyone who follows the data science world knows that there are many solutions, all of which are quite good in their own way. Organisations embrace platforms for managing models, for example, have data lakes in AWS S3 and Azure Blob Storage; we can go on like this for a while. Today, a modern organisation can have dozens to hundreds of data science-related solutions in use. However, all these different frameworks and tools lead to more complex architectures.
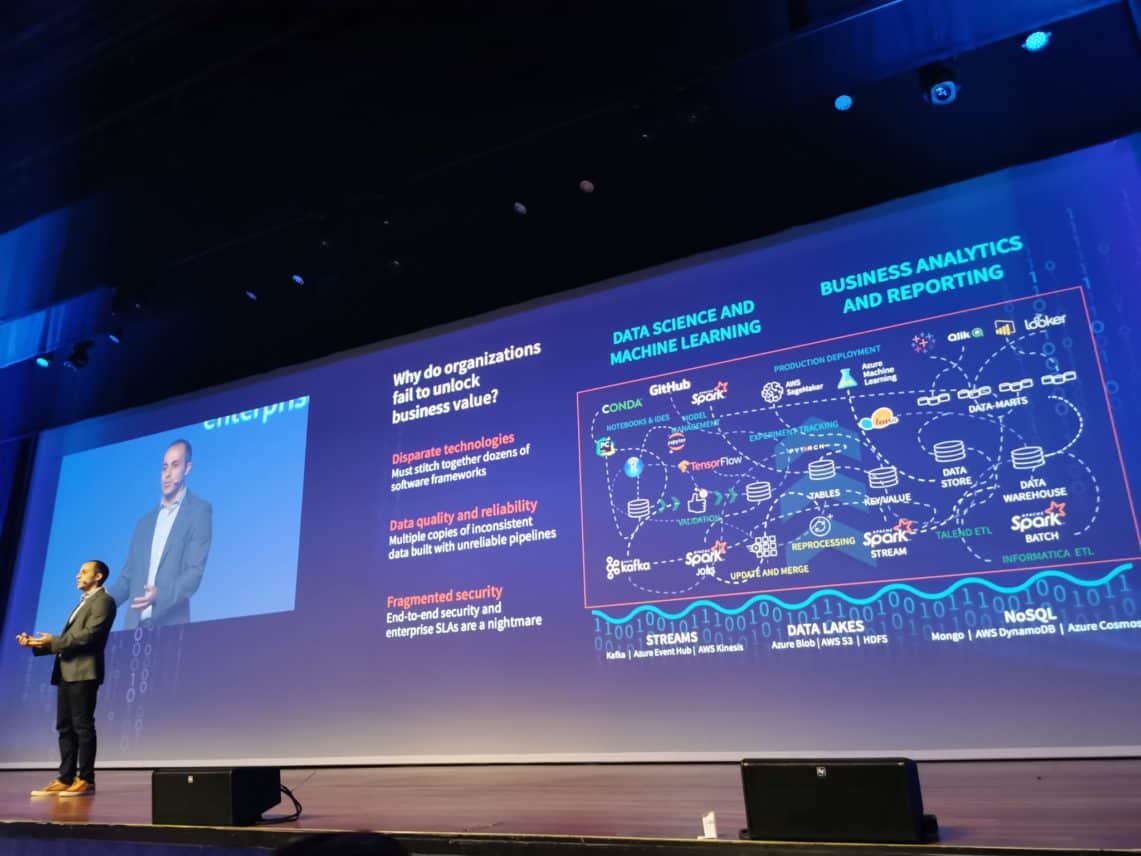
At Databricks, one finds that the more complex infrastructure means that data is now an issue that concerns many different professional groups. DevOps teams, for example, have to configure all the separate components. Everything must work and preferably also communicate with each other. The software must be configured and updated so that it all complies with modern standards.
In addition, an extensive infrastructure means that it is difficult to continue to guarantee data quality. According to Armbrust, this is because there are multiple copies of inconsistent data resulting from unreliable data pipelines. “Data science has, therefore, become a matter of data maintenance, primarily,” says Armbrust. “As a result, data scientists work far less on their actual tasks.”
Finally, security teams are also burdened by complexity. Taking more solutions into use will allow the IT environment to grow. This larger IT infrastructure and the growing size of data lakes increase the area of attack. This is something that security teams have to take into account, because in the event of a data breach, they are blamed first.
The effect of Apache and Delta Lake
The platform of Databricks consists of multiple layers to address these problems. The first component it uses for this is Unified Data Service. With this layer, data quality must be guaranteed throughout the entire data journey. To this end, the platform offers management options for data storage, processing and ingesting. The Spark and Delta Lake frameworks have to support this.
Spark was originally developed during a university project, and a bit later it was decided to set up Databricks. The potential they saw was a significant performance improvement in ETL (extract, transform, load), compared to the standards at that time. The analytics engine extracts data from all kinds of sources, from disk storage to EC2. Spark then uses various resources, such as in-memory computing, to quickly process data sets. Developers realise performance improvement with different features. For example, they can configure a custom cluster. ETL, however, remains the message that best describes Spark.
“Data science has become a matter of data maintenance, primarily
Delta Lake is the other framework of the Unified Data Service layer. Compared to Spark, Delta Lake may be less well known, but that doesn’t make the component any less important. The framework runs as a storage layer on top of the data lakes of organisations. In this way, it can store data in such a way that frequently used technologies can read out the data. It becomes easier for organisations to extract value from data, but they can also modify and delete data more quickly.
Layer to promote collaboration
With another component, the Data Science Workspace, the Databricks platform aims to promote collaboration on data and machine learning projects. This is a dashboard for managing analytics projects.
At its central location, Databricks maps the artificial intelligence (AI) models of organisations. Such a central point is useful for sharing the models between data scientists so that together they can ensure that they can actually be put into use. Security can be built-in, updates have to be easy to implement, large datasets are easy to store in cloud services and more. Databricks wants to add enough extra features on top of the open-source version so that all the wishes that come with model management are fulfilled.
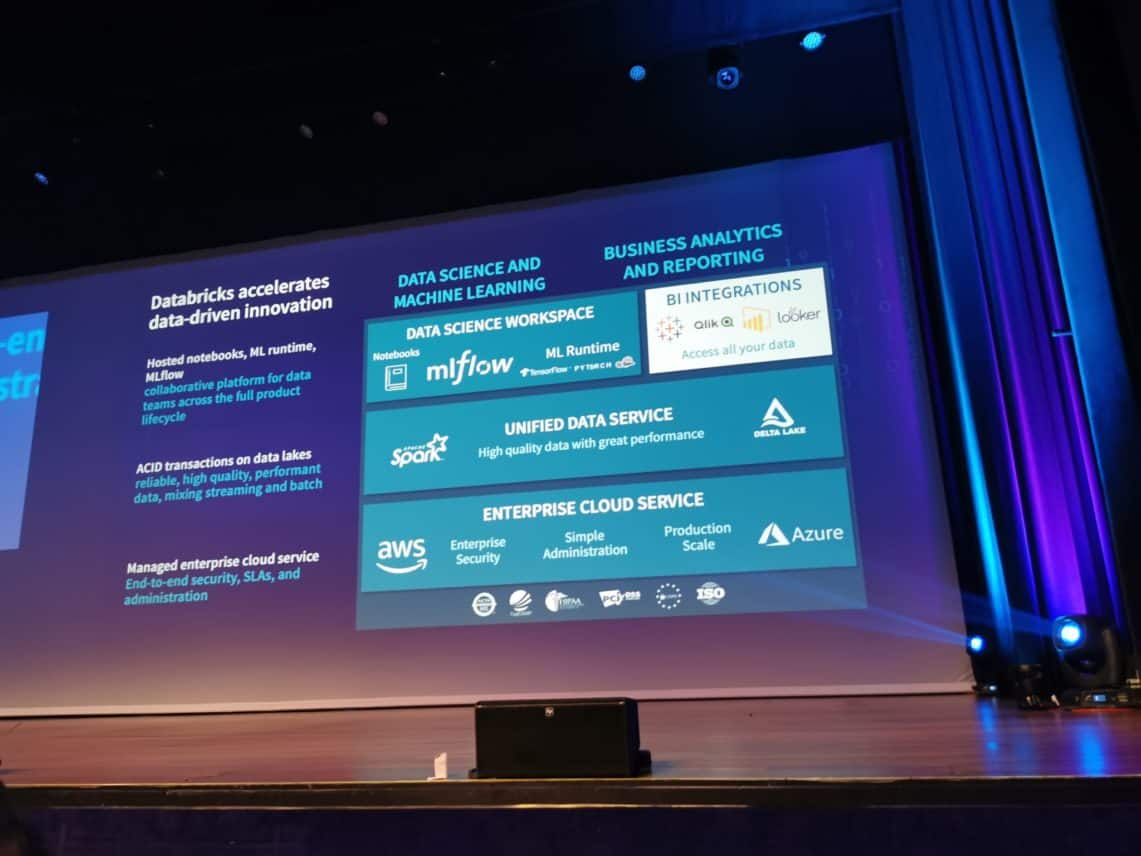
Open-source is necessary, according to Databricks
So, the Unified Analytics Platform consists mainly of open source software, which is what Databricks really believes in. There are some concerns about the profitability of open source, but this does not seem to phase Databricks that much. After all, opening up the source code to other developers accelerates the development of the frameworks. The developers are given the freedom to experiment with the software, which means that the user application can change from what Databricks originally intended. Perhaps the functionality will evolve in such a way that data science becomes a little more accessible. The innovation brought about by open source is something that Databricks very much encourages.
In addition, Databricks, as the original creator, knows the frameworks very well. Because of this, it knows exactly which premium features it can add on top of the software, so that the frameworks are of extra value to the platform. Companies appear to be very enthusiastic about this, because the adoption of the platform, for which of course payment has to be made, continues to increase. This way, Databricks earns good money, innovation is promoted, and companies get a platform that promises to simplify data science.
Intended as a simplification, not as a complete replacement
Databricks therefore particularly wants to simplify matters, in which an open-source approach is seen as crucial. However, the company also considers it important to be able to exist alongside existing solutions. After all, you don’t solve a problem on your own. Databricks supports the use of its own tools in combination with other solutions, by setting up integrations with other analytics and AI solutions. Databricks continues to work on this. However, the fact is that a combination of Databricks with frequently used Business Intelligence tools should not be a problem right now. Connectors have been built for Tableau, Microsoft Power BI, Google Looker and Qlik so that data can be retrieved from Databricks clusters. Furthermore, there are deep integrations with tools such as Dataiku and AWS SageMaker, while it can also be used alongside commonly used solutions such as SAS.
All in all, it is quite logical that a relatively young company like Databricks attracts the attention of several large enterprise organisations. The company has found an innovative approach to help organisations with their analytics and AI projects. As the scaling up of such projects is in full swing, new solutions can be a simplification that organisations are waiting for.
We are, therefore, curious to see how Databricks will develop in the coming period, and we will continue to keep a close eye on the company.