In May 2018, the introduction of GDPR (General Data Protection Regulation) forced organizations to rethink the way they collect and store data. However, even years after the legislation went into effect, organizations still seem to be struggling with their data and lack proper fundamental data governance. We see a big discrepancy between the potential that lies in data, analytics and decisioning on one hand, and the reality in the data field on the other. Too many organizations are still knee deep in the data mess.
The challenges that organizations are facing with data governance today are very much the same as they were fifteen to twenty years ago. Effectively accessing data, making it available to the business to drive innovative use cases, to digitalize business processes with automated decisioning, is proving much harder than it should. Why? Because the data is still very much an issue. There is a huge mismatch between the aspirations of organizations to become data-driven and the reality of their day-to-day struggles. In many cases, the move to hybrid cloud architectures and the creation of cloud-based data stores have added to the confusion.
Even within the most advanced organizations with a strong data culture, the majority of data professionals is struggling with basic requirements around data access and data quality. Off-line, uncontrolled, spreadsheet-based data manipulations are still the norm. Armies of data professionals are involved in repetitive, low value and pointless tasks, and too often without a clear purpose. The waste of talent and resources is a missed opportunity to leverage the data to create value.
The Data Analytics Marathon
Early in 2022 Forbes published a piece by author Brent Dykes ‘Data Analytics Marathon: Why Your Organization Must Focus On The Finish’. Dykes describes data analytics as a long-distance running race in six stages, from data collection, followed by data preparation and visualization. After that comes analysis, insight communication and finally it is time to take action and deliver value.
The percentages in the diagram represent Dykes rough estimates for how many companies reach each milestone in the data analytics marathon. According to Dykes the vast majority of time and effort is spent at the initial stages of the race. Trying to sort out and fix the data. Very few organizations at the start manage to actually finish the race. That is to get to the point where they deliver value to the business. Many organizations are exhausting themselves in the early stages and never reach the interesting bits: predictive analytics and decisioning.
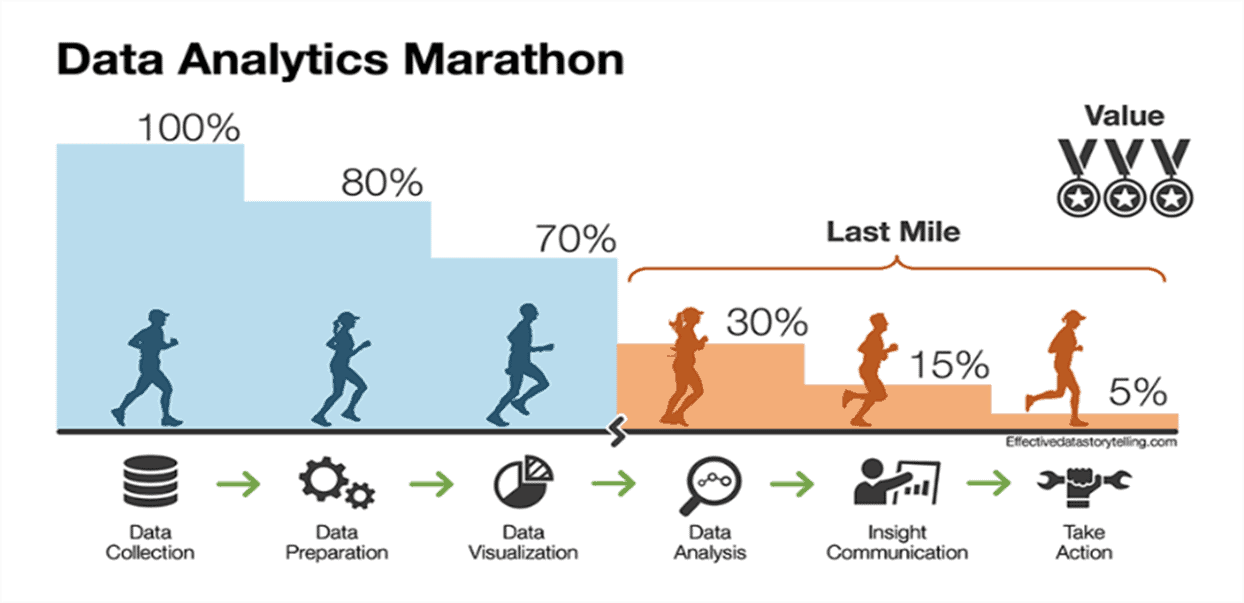
Start from the finish line
The question is: How can we run this race differently and more effectively? How can we bring some balance into this and help organizations get to the finish line? Our recommendation: start the data analytics marathon at the finish line. If you know what the finish line looks like, or where the finish line is, you can work backwards to the start and make sure you focus on data challenges that really matter to the business outcomes. Ask yourself: what do I want to do from a business process perspective? What decisions do I need to make as part of the customer engagement? What insights or predictions do I need to make those decisions? What data do I need to conduct this analysis?
Instead of ‘boiling the ocean’ (or maybe we should say: the data lake) and trying to fix all the data, you should create a value chain between the decision you want to make or automate, and the data that you need to do that. If you want to upsell something to a customer, real time and across channels, what kind of insights do you need? Knowing what you need, helps prioritizing the requirements for your data science and data management and governance efforts.
TIP: SAS forges the future of analytics in the cloud
Consumption driven data governance concept
To help organizations achieve this, we have developed a framework to map these end-game decisions or business outcomes to data requirements. We call this framework “consumption-driven data governance”. By using this approach, organizations can narrow the funnel and focus on what really matters, giving them the possibility to refocus their efforts on value-adding data consumption activities.
We see too many organizations embarking on a journey to “fix the data first”, but in reality, this is never going to happen. You don’t have to fix the entire data landscape and bring enterprise data governance to it all. Years of trying did not help. What you need is to target specific usage consumption.
To make that happen you need a holistic data and analytics strategy, instead of separate strategies made by a chief data officer, a head of analytics and the head of lines of business. There is no point in developing a data strategy if it is not aligned with the business outcomes. We advise customers to really embrace this end-to-end value chain and to make sure they create alignment between the different stages of the race. A good deal of the time and effort spent by data professionals in the early stages can be automated with data pipelines. AI-capabilities can help in the continuum of data preparation, cleansing, visualization, analytics modeling and decisioning. That will accelerate efforts in the early stages and help your organization in focusing on added-value insight creation and automated decision-making.
The data marathon is an accurate metaphor, but we think the data analytics race is more like a relay. Collaboration is critical; it is never a one-man job. The baton should be passed on from the data owners or producers to data engineers and business analysts and to data scientist or even decision scientists. They must be able to look at the same data in the same environment all using their own specific skills, views, tools and preferences. Collaboration is key to reach the finish line.
This article was written and submitted by Olivier Penel, Head of Global Advisory at SAS and Rein Mertens, Head of Customer Advisory, SAS Platform