IBM claims that watsonx provides the building blocks for successfully deploying Generative AI initiatives in enterprise environments. What are these building blocks and why is IBM so confident that they can do this better than others in the market? We recently attended the first edition of IBM TechXchange EMEA to learn more about this.
IBM announced watsonx last year at its own Think conference and within a year has built it into an enterprise AI stack. This makes it possible to deploy and deploy GenAI in enterprise environments. That is, the platform includes all the components important to making this happen: watsonx.ai, watsonx.data and watsonx.governance. The first two were immediately part of the stack at launch; the third was added in November 2023.
Components of a successful AI strategy
Organizations can purchase all of these components from IBM, or they can choose only the ones they need. However, it is important to set it all up using a “recipe for success for AI in enterprise,” as Dinesh Nirmal, SVP Products, IBM Software put it in his keynote during TechXchange.
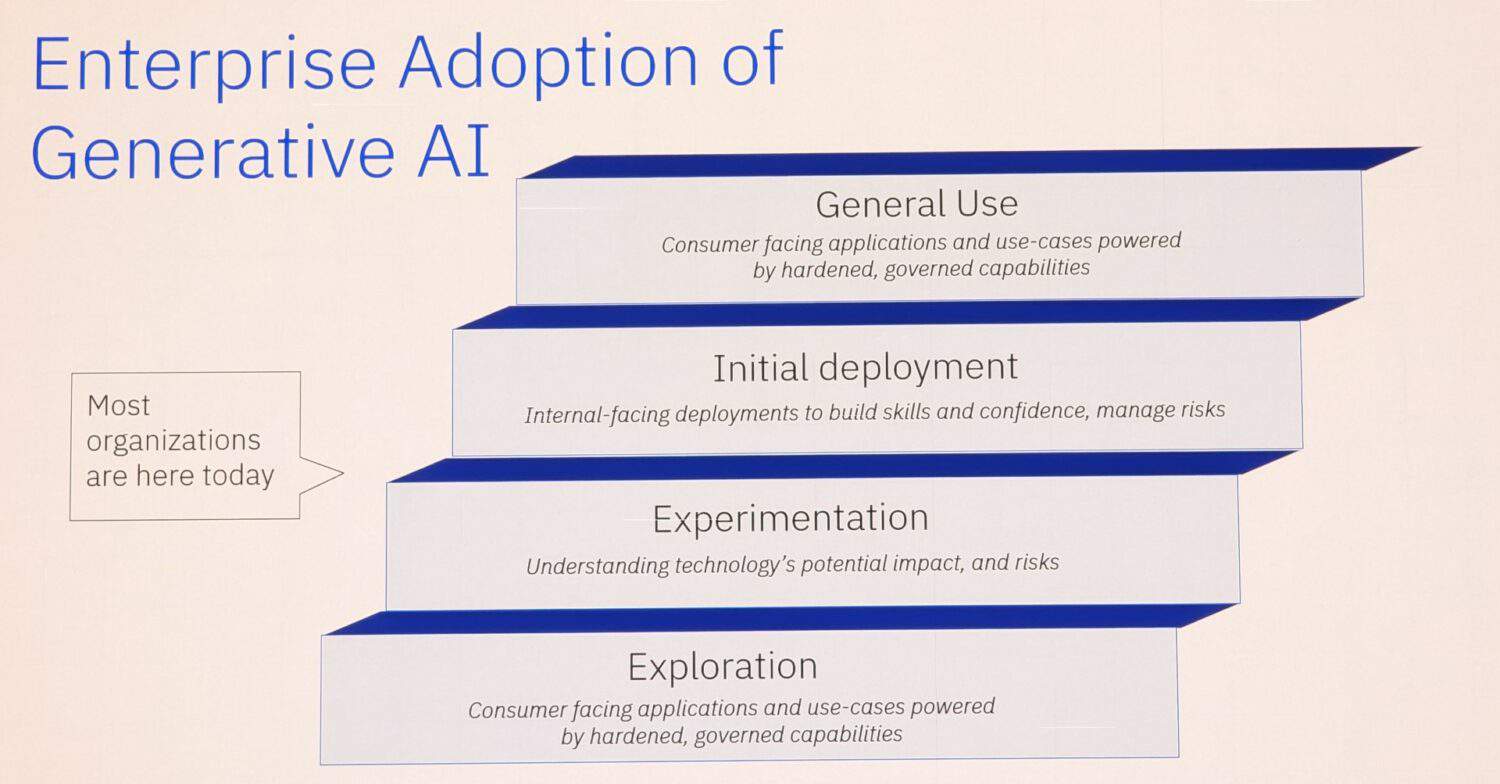
Integrated platform
The recipe Nirmal is talking about consists of three ingredients. The first is a tightly integrated platform, or AI stack suitable for enterprise environments. At IBM, that’s watsonx. Nirmal makes a point that this is not a closed stack. There is an SDK available and you can use (REST) APIs. He sees generative AI not as a world where there is only one winner, but where anyone can be a winner.
That anyone can be a winner sounds a bit too positive as far as we are concerned, although we understand what Nirmal means. There will not be one single model, vendor or approach that will win out. When we speak to him briefly 1-on-1 after his keynote, he prefers to talk about a set of highly integrated technologies rather than platforms. The latter always has some single-vendor associations. IBM can, of course, provide the necessary expertise in all areas, should customers want that. We will come back to this later.
AI models for enterprise purposes
The second ingredient consists of the AI models being used. These must be suitable for use in enterprise environments. IBM has its own foundation model, Granite, but also, for example, a partnership with Hugging Face, which allows organizations to use open source models. This open approach regarding models is necessary because there is no one model that is suitable for all uses.
Nirmal also points out that it is not (always) necessary to use a model with 70 billion parameters. With fewer parameters, you can do an excellent job as well. Fewer parameters will most likely produce a slightly less accurate result, but that is not necessarily a bad thing. For some use-cases, extremely high accuracy will be more important than for others. Smaller models will also bring down cost. That is not insignificant, Nirmal observes: “Cost is an inhibitor.” From that perspective, he sees a clear trend: “Models are getting smaller, more focused and domain-oriented.”
Transparency
The third ingredient Nirmal talks about is openness and transparency. We actually saw this reflected in the first two as well. Here, however, Nirmal is mainly talking about the openness around training models. IBM, he says, is the only vendor that publishes the data it uses to train models. With other models, it is often not even clear where the training data comes from, he argues. So they can’t even provide that openness. Especially as things like copyright violations come under increasing scrutiny, it is important that models are explainable. Part of that is making it clear where generative AI models get their training input from.
GenAI is not optional, ROI is clear
Nirmal indicates several times during our conversation that GenAI is still in its infancy. Still, this is no reason for organizations not to get started with it, he points out: “If you don’t do it, you’re going to get left behind.” Consequently, he sees most companies looking at GenAI to determine where it can add value.
This doesn’t mean that those companies are actually using it yet, by the way, in part because of the still fairly high cost involved. However, the ROI is clear, Nirmal points out. In particular, RAG, or Retrieval-Augmented Generation, has taken off in 2023. RAG means that LLMs have access to a knowledge base that allows it to ensure that answers are as accurate as possible. So it doesn’t get this extra knowledge from training data, it looks for it outside the training data. You can think of it as the difference between extracting extra knowledge from a book by flipping through it and retrieving information directly from one’s own memory.
RAG is without a doubt the most important application of GenAI for organizations right now. In addition, there are others. These include things like content generation (including by developers who want to write code faster) and text summarization, as well as generating insights from larger and diverse data sets.
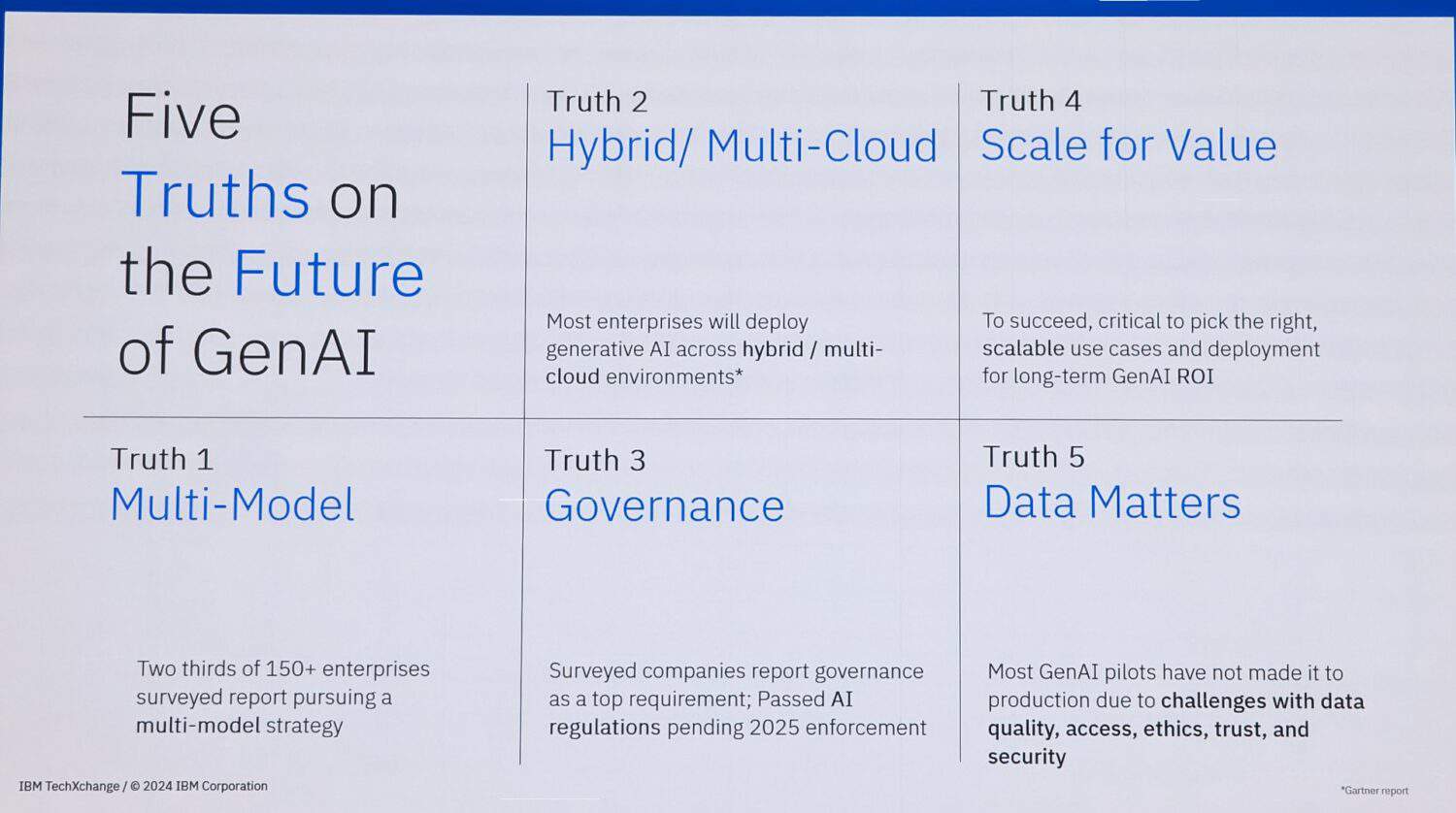
Moving forward step by step
As already indicated, GenAI for business applications is still in its infancy. However, Nirmal dares to make some predictions about its future. He gives a total of five, which we list below:
- Organizations will continue to opt for a multi-model strategy.
- GenAI will be rolled out in a hybrid cloud model.
- Governance will become one of the key requirements.
- Organizations must choose use cases that are scalable. Otherwise, it’s impossible to extract sufficent value from GenAI in the longer term.
- The importance of the data that organizations use will only increase.
Of the five predictions, we highlight 2, 3 and 5 in particular. Hybrid is the norm, and will be the norm for the foreseeable future. This makes environments as a whole more complex, but also means that implementing GenAI can be very complex too. “Model training itself already makes it complex,” Nirmal points out. Also, in organizations in certain industries, data may not go outside the firewall. “And how do you train a model for a customer with sites in 30 different geographic regions,” he asks out loud. Mind you, we’re not even talking about deploying it in hybrid environments yet, just the training of the models. Using GenAI in hybrid environments brings with it several other issues, including governance.
IBM has a lot of experience with governance
IBM puts a lot of emphasis on watsonx.governance. This is not surprising, as it is the latest addition to the AI stack the company has developed. In addition, governance is also something IBM is working on a lot anyway. The company has been active in data governance for decades. The move to AI governance is then relatively easy. Nirmal is clear about this: “No vendor does governance better than IBM.”
Governance is undoubtedly important in a GenAI strategy. However, the main reason GenAI pilots do not go into production has to do with issues around data. Data governance also plays a role here, of course, but there are also often problems with data quality, or access to the data. Organizations need to organize that properly first. “If you don’t have a data strategy, there’s no AI strategy,” Nirmal clearly states.
GDPR, by the way, has provided a positive development in the area of data strategy. To comply with it, organizations had to get their act together around data. So does this mean that European companies have an advantage in rolling out GenAI? Nirmal doesn’t want to go that far. However, European companies do have to be much more methodical because of the GDPR. He also immediately adds that American enterprise organizations also manage their data very well.
Conclusion: clear and transparent framework should make complexity manageable
All in all, GenAI poses quite a few difficult questions to organizations, if they want to roll it out in a good and thoughtful way. It is important that organizations think about this carefully before they start. In our opinion, the steps proposed by IBM are a good framework and a good start, especially since it is basically an open approach. IBM will no doubt prefer to sell as many of its own building blocks to customers as possible, but it is not blind to reality either. That is always nice, because this reality is quite complex and GenAI only makes it more complex.