Databricks, with its lakehouse, has spent the past few years establishing a strong architecture for the AI workload. Now that that story stands and is being further optimized, Databricks is working to better serve the analytics workload. From CEO Ali Ghodsi, we understand that companies are responding enthusiastically, and the outlook is bright. What exactly is the strategy?
With the lakehouse, Databricks has grown into a company that generates more than a billion in revenue within a few years. This platform, which unifies structured and unstructured data on a single architecture, is why most organizations use Databricks. The lakehouse uses open-source components and allows companies to manage data from both traditional data warehouses and modern data lakes. In this way, it simplifies access to and use of different data types and it allows to run data workloads on the same infrastructure. The platform mainly thrives when running AI workloads.
Mosaic to optimize GPU usage
At the recent edition of the Data + AI Summit in San Francisco, it was apparent that Databricks’ Data Intelligence Platform remains full of development as the basis for AI workloads. This makes sense since the needs around AI are also constantly changing. A basic architecture like Databricks needs to be constantly responsive to that. The most significant investment in this area is from Mosaic. Databricks acquired this technology last year. Mosaic can offer many extras when it comes to supporting AI workloads.
Such an addition was attractive for the platform because while the original Databricks technology can optimise CPU usage, it cannot do so much with GPUs. CPUs are useful when training models without too many resource needs, such as machine learning for predictive maintenance. However, if you train a model that requires a lot of processing power, it makes more sense to use technology to optimize GPU usage.
The GPU is stronger at training advanced models, but energy-heavy GPU power should be as efficient and economical as possible. Hence, with Mosaic, technology is now available to optimize GPU usage.
Also read our story in which we discuss all the Data Intelligence Platform has to offer.
Biggest change for Data Intelligence Platform
Of course, using GPUs through the Databricks platform was already possible, but the possibilities are now more extensive. In addition, through Mosaic, Databricks adds an engine to the Data Intelligence Platform that applies automatic indexing and data partitioning. It also adds descriptions and tags to data assets in the governance solution Unity Catalog. This strengthens semantic search and improves the quality of AI assistants.
In addition, Mosaic is bringing technology to the Data Intelligence Platform to help build AI apps. For example, at the Data + AI Summit, the public preview of Mosaic AI Model Training was unveiled, which enriches open-source models with enterprise data. This results in more reliable and cheaper models. The Mosaic AI Gateway provides an interface for managing and deploying models and monitors cost and security. Mosaic AI Vector Search and Mosaic AI Agent Framework are also coming to develop and evaluate RAG (Retrieval-Augmented Generation) applications. In this way, the accuracy of LLMs should improve by using multiple interactive components.
Tip: Databricks solidifies Mosaic AI as a foundation for building AI apps
Where does analytics stand now?
This entire optimization of the Databricks platform for AI will ensure that with the expectations around artificial intelligence, the company has a solid foundation to continue growing in the coming years. The lakehouse and now the Data Intelligence Platform have ensured that Databricks is the default choice for multinationals to run AI workloads. Those same multinationals often also run legacy data warehousing products and more modern platforms to run analytics workloads. That results in multiple data architectures from different vendors with their own goals. Couldn’t you reduce that number to make the data foundation work more efficiently?
So, we asked Ghodsi about the progress made in the analytics field. That’s where Databricks has been much more active for about four years. Initially, through the private preview of Databricks SQL to bring data warehousing capabilities and SQL support to the lakehouse, followed by general availability in late 2021. This will allow companies to run business intelligence (BI) and reporting better on the platform. Ghodsi indicated that Databricks SQL is now the fastest-growing product in Databricks’ history. In that time, $400 million in annual revenue has been realized, which amounts to some $373 million. In contrast, Databricks is also behind projects such as Spark, Delta Lake, Unity Catalog and Koalas. All products with a sizable presence in the data world, but which thus did not initially grow as fast as Databricks SQL.
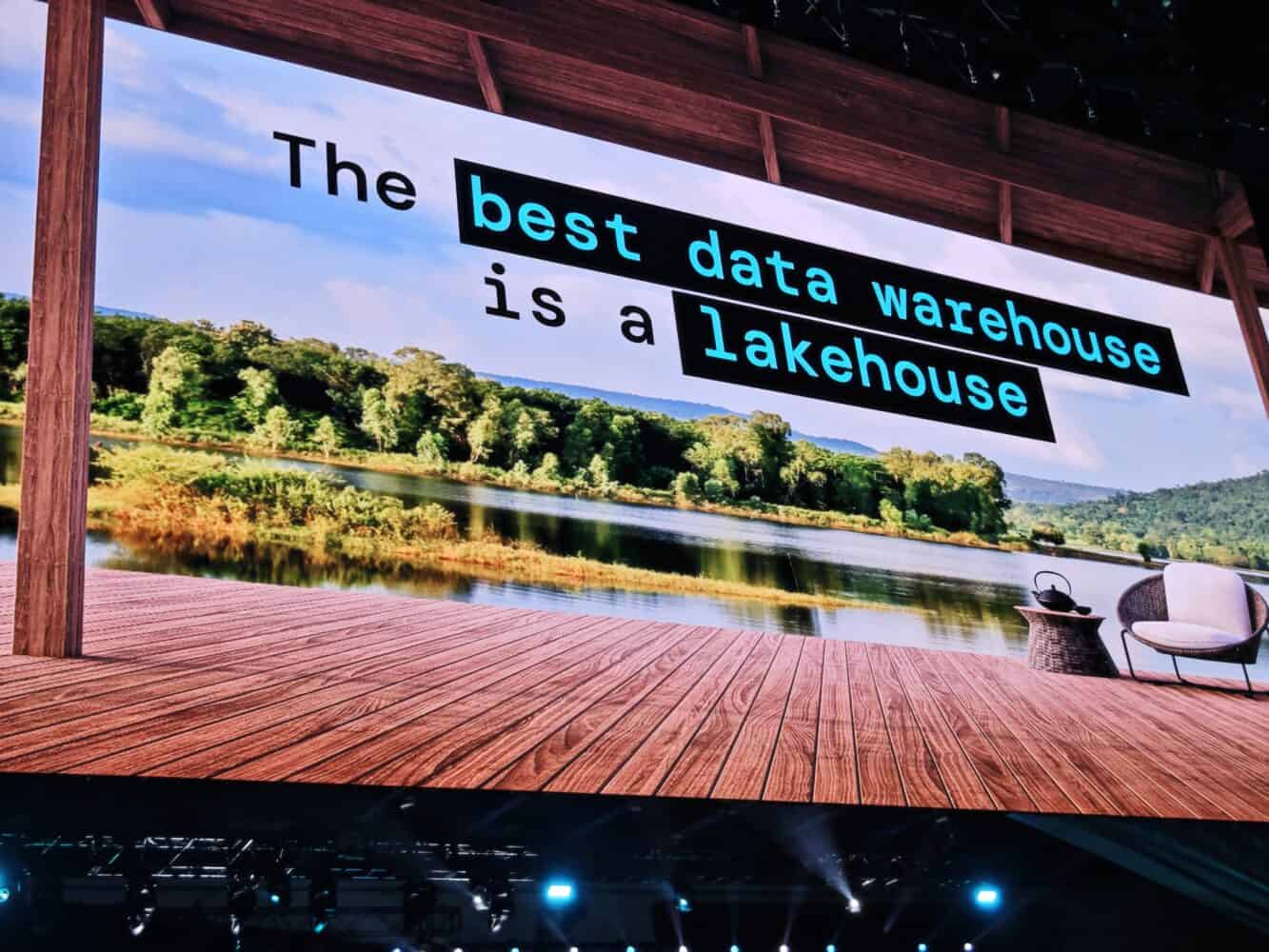
Being ten times better than the rest
Ghodsi indicates that at launch, something had to be done differently with the analytics product to make it a success. After all, Databricks did not yet have a very good data warehousing product during launch. So, one of the founders of Databricks, Reynold Xin, started looking for qualified personnel to set up a capable team. “We didn’t just say: ‘We’re gonna build a data warehouse, and it is good enough. Trust us that it is as good as what is out there.’ Then you can’t really win that one. So you have to be ten times better if you wanna win,” Ghodsi said.
With those last words, the CEO is referring to the data warehousing benchmarks that appear regularly. In those, Databricks SQL beats competitors by a factor of 10+ when it comes to price performance. “We disrupted it by saying it is not a data warehouse. It is your own data, you own it yourself. No more proprietary lock-in. And you can do AI on top of it. So, we had a disruptive approach to it, which allowed us to grow it so fast,” Ghodsi adds.
Tip: Unity Catalog is now open source software
What are the plans for analytics?
Now that Databricks has gained a foothold in the analytics world, there are plenty of ambitions to grow further. That’s why the company built the new BI product Databricks AI/BI. This is designed to understand data semantics and helps employees analyze data themselves. Ambition to get business users more involved in BI has generally not been lacking in recent years, but the basics are still mostly the job of data experts.
Databricks AI/BI is based on a compound AI system, meaning tasks are handled by combining multiple interactive components. AI/BI uses such a system to extract insights from the entire data lifecycle within the Databricks platform. The components that AI/BI takes with it are ETL pipelines, lineage and other queries.
Through this compound workflow, AI/BI enables two things. First, the AI/BI Dashboard is a low-code dashboarding solution with all the conventional business intelligence capabilities for answering queries. In addition, there is Genie. This feature involves a conversational interface that continuously learns the underlying data and semantics based on human feedback. Genie can answer a broader range of business questions based on its reasoning capabilities while still delivering certified answers for query patterns specified by the data teams.
With these investments, Databricks shows its desire to shake up the analytics market after first changing the AI world. In time, we will have to see if the amount of warehousing and other data platforms decline, but the first signs are visible. We look forward to seeing how Databricks’ AI and analytics approaches develop.