There is no shortage of ambition at Red Hat. The company offers a range of tools for model training, Red Hat Enterprise Linux AI and Red Hat OpenShift AI, all to enable open enterprise AI. In conversation with Jeff Lo, Vice President Portfolio Marketing & Learning, we take a closer look at the possibilities.
As far as Red Hat is concerned, open enterprise AI is the way to get your artificial intelligence strategy right as a company. Companies are exactly now looking for ways to improve business with AI. Yet AI is not a completely standalone event, Lo argues. Rather, we should see it as an evolution of previous innovations, such as the cloud. There, an open approach has proven itself. That can largely be extended to AI. It means open-source, adaptability for any infrastructure environment, a strong ecosystem and the ability to integrate with different systems.
Open-source should be common for models
When it comes to the AI boom, Red Hat can rely on its experience, which goes back to the 1990s, when it made its name and fame with a Linux distribution. It took on proprietary software to bring open source to the data centre. Even then, it became clear that Red Hat sees an open approach to software creation as the preferred way of working. With all the new tech waves – cloud, edge, hybrid cloud – this open source philosophy has remained intact. So now we are moving towards open enterprise AI.
We should note here that fully open in the AI era is easier said than done. Some models present themselves as fundamentally open. However, reality shows otherwise. They may be free to download, but it is then impossible to trace the origin of training data and tweak performance. ‘We want to make open source-licensed models as common as software. Then everything will be open and available, and communities will work on it together,’ Lo said.
Which models work?
As we also open this article, Red Hat already has solid capabilities at its disposal to enable that open enterprise AI. For enterprises, however, it is not readily apparent exactly how to approach AI. This is due to several challenges Lo observes. First, companies often do not know which model to choose. Initially, a single LLM seemed the logical choice because of the capable nature they showed. At the same time, implementing dozens or hundreds of models also seems like an interesting option, because then there is an AI model available that is very suitable for a specific scenario. The latter choice is actually preferred in previous IT scenarios: from software solutions, databases and clouds, large companies embraced multiple options, due to cost, manageability, flexibility, data privacy, and other reasons.
Besides the burden of finding the right models, companies are faced with the financial picture. How will you ensure that costs remain manageable? You achieve a powerful model with lots of data, training, refinement and optimisation. All parts that require computer resources and man-hours. You can then try to estimate how much a single model will cost, but will you stay within that budget? And is that investment commensurate with the efficiency gains from the model? One way to overcome these obstacles is to opt for smaller models.
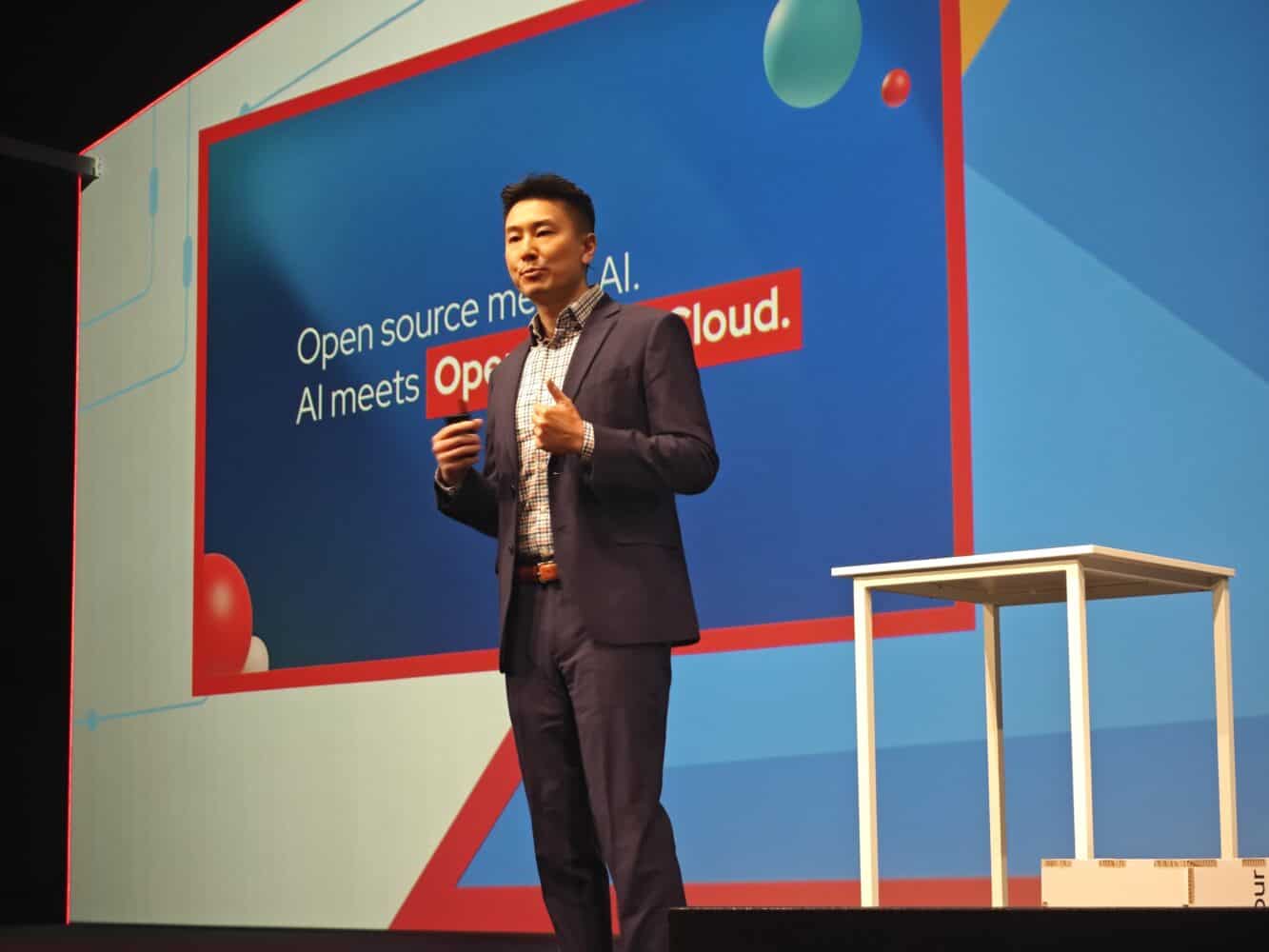
Third, there is a final consideration when completing the AI strategy: finding simple tools that can be widely used in the organisation. Suppliers of data and AI platforms are constantly looking for ways to respond to this desire. This desire stems from the fact that companies simply do not have enough data scientists to develop, curate data and train enough models. One possible solution is then to provide simple tools that business users can use. They also have knowledge of their domain, so they know which data is important for the model.
AI in business operations
To address these challenges, Red Hat has introduced completely new technology and expanded existing offerings. The former is the case with InstructLab, a project that saw the light of day in May. The goal? Getting powerful models with a smaller footprint into the hands of more employees. They work together within InstructLab to train a model. Importantly, InstructLab also lets a company use its own data and run the model in its own private environment. A guarantee of security and control over the data. All this in such a simple way that training models does not require years of training as a data scientist. From experience with an earlier short demo, we can also say that the environment works quite easily.
Tip: What is the new AI project Red Hat InstructLab?
The smaller models built with InstructLab can make operations more efficient, but there is also demand for off-the-shelf LLMs. For this, Red Hat has tapped parent organisation IBM. Traditionally, IBM’s mainframes have been widely used for AI workloads, which has helped lead to the research department doing a lot of research on AI. From these researches came the Granite family of LLMs. Meanwhile, Red Hat offers Granite plenty following the open source philosophy. That is, insight into where the data comes from, how the model is built and an open licensing structure. Through Hugging Face and GitHub, these off-the-shelf models are available.
The core: RHEL, OpenShift and Ansible
Red Hat actually supports enterprise infrastructure by default with three core products: RHEL (Linux), OpenShift (Kubernetes/containers) and Ansible (automation). These three products remain unified even in the AI story. Indeed, Red Hat sees AI as an application that you should treat largely the same as software and for which the principles are virtually the same. In other words, run a model where you want, scale up and down when desired and run third-party workloads.
In this, Lo sees RHEL AI as the easiest stepping stone towards getting started with generative AI. The dedicated RHEL version works with the Granite-LLMs and InstructLab, by running these tools on a server via an image. In this way, it is possible to make use of company data located in the data centre or the cloud. RHEL AI works optimally through collaborations with Nvidia, Intel and AMD. There are also bespoke options on the server side. The big three – Dell, HPE and Lenovo – enjoy support.
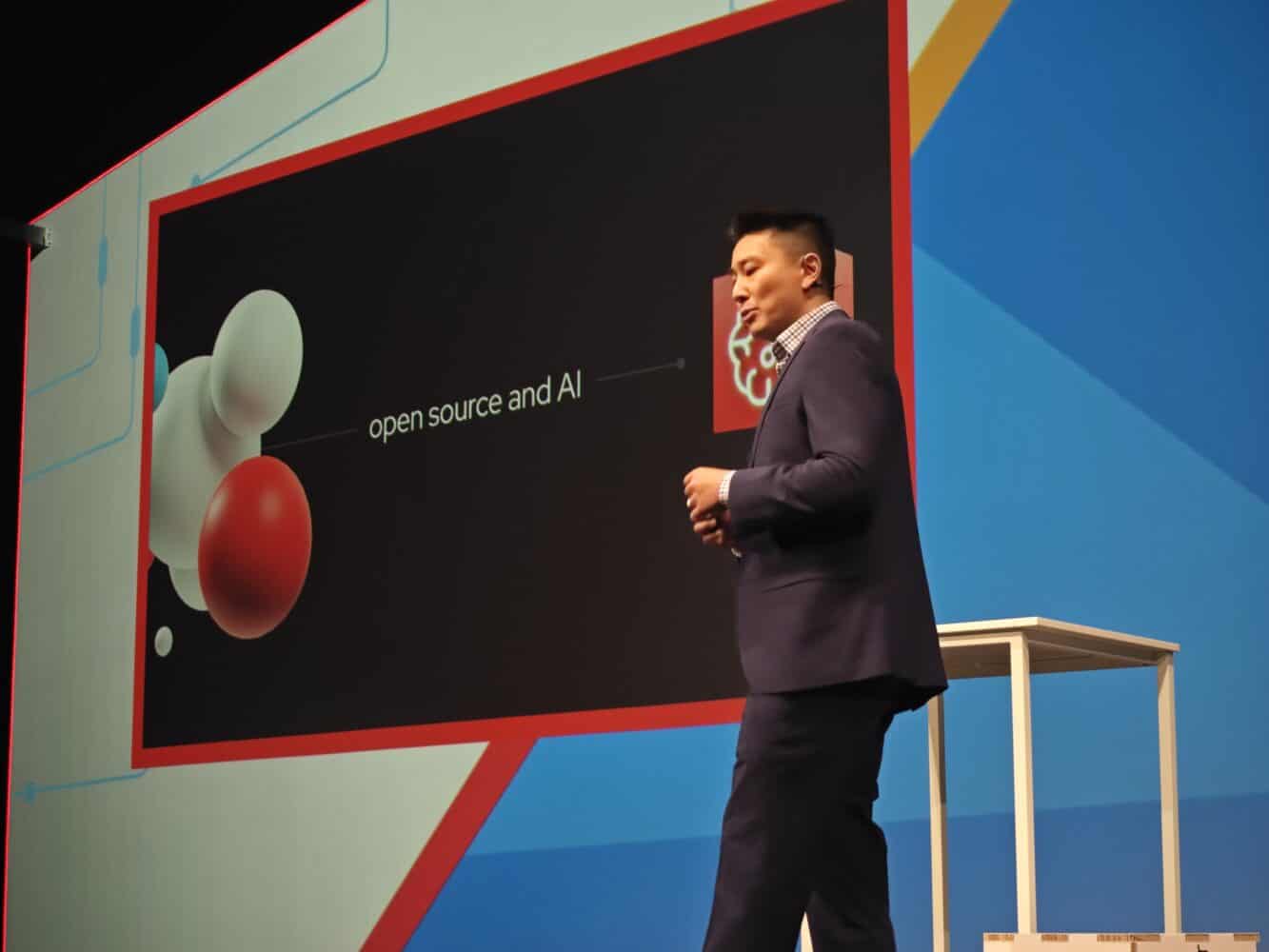
If you want to deploy and scale AI applications, that’s where OpenShift AI comes into the picture. OpenShift AI includes RHEL AI and makes it easy to deploy AI workloads in hybrid and multicloud environments, as well as providing options for managing the containerised workloads. It also comes with an MLOps platform in which options for model management and automation can be found. These include components for model development, monitoring and automated training. Companies that choose OpenShift AI will automatically get RHEL AI with it.
To really complete the infrastructure for AI, as far as Red Hat is concerned, Ansible Automation Platform is still crucial as a consistent foundation for automation and collaboration across the organisation. As the number of AI applications in the infrastructure grows, so does the number of events and insights generated. If you want to keep responding to these, enterprise-wide automation is almost indispensable. By writing playbooks with Ansible Automation Platform, the right actions can be triggered automatically.
Tip: Event-Driven Ansible will unleash a new wave of automation
Towards broad adoption
Prior to our conversation with Lo, we also attended a presentation by US children’s hospital Boston Children’s Hospital. The presentation by Dr. Rudolph Pienaar, Technical Director, Fetal and Neuroimaging and Development Science Centre, made it very clear how open AI is applicable. The hospital’s radiology department takes brain scans of young patients. Getting good radiographs of children can be a challenge in itself, especially in babies whose movements are unpredictable. However, doctors need a still image to make the very best diagnosis. With the ambition to improve the image quality and accuracy of image interpretation, the hospital started looking for innovations with AI.
Software tools for improving the image quality of medical images have been around for a long time, but are cumbersome to use and can take a long time to run. The hospital decided to explore using AI to speed up this process. In fact, AI is very good at this. If you have something that already exists, AI can “mimic” it accurately and often this mimicry runs much faster. With an internal research team, the hospital built a new platform called ChRIS that can run these AI apps and others. ChRIS runs on top of Red Hat OpenShift, and so these apps can be run more efficiently. For instance, an AI app on ChRIS/OpenShift can automatically look at children’s X-rays to find data points that indicate a possible abnormality or, on the contrary, signal that everything is fine. When such a model is trained on thousands or hundreds of thousands of photos and data points, it knows exactly how to diagnose almost flawlessly.
While AI apps are important, the platform that allows them to run is just as important. Boston Children’s Hospital therefore has ambitions to bring both the ChRIS platform on Red Hat OpenShift and all the AI apps it runs to more hospitals. An open approach in keeping with Red Hat. The tech company likes to facilitate such social projects, although the open nature is also something that can have a wider impact in more commercial situations. Red Hat at least has the foundations in place for this. It is now up to companies to further embrace open enterprise AI.