Everyone who does anything with cybersecurity will no doubt recognize the statement “it’s not a matter of if, but when”. At Deep Instinct, however, they fundamentally disagree with that statement. According to this supplier, deep learning is the way to go from reactive endpoint security back to proactive, i.e. preventive endpoint security. What do they mean by that? Read on to find out.
We are bombarded from all sides these days with stories about new and groundbreaking solutions in the world of cybersecurity. It also seems as if new security vendors are being added daily. The result is a very crowded market, with thousands of suppliers for organizations to choose from.
Of course you can interpret such a proliferation of providers as a signal that there is simply a lot to do in the world of cybersecurity. So it makes sense that there are many suppliers. If you have a more cynical (or realistic) view of life, you could also say that it is apparently difficult to put a product on the market that is so good that there is no room for too many other players. So as an organization, you keep investing in new solutions because what you bought a while ago doesn’t protect you adequately.
One of the reasons that previously purchased security products no longer work well is that the working environment has changed very rapidly and is still changing. In particular, the fragmentation of an organization’s (IT) environment plays an important role in this. The statement from the introduction actually refers to this as well. Where we used to focus mainly on prevention, with firewalls and antivirus, that no longer seems tenable. The perimeter is no longer the firewalls, but more or less everywhere, with all the endpoints in your organization. In effect, that means there is no perimeter anymore.
The same story everywhere
The shift from prevention to detection is also cited by Rob Huikeshoven, Senior Sales Engineer at Deep Instinct, in conversation with us. He hears the same story coming up almost everywhere: Organizations initially made investments in firewalls, Anti-Virus and even Next-Gen Anti-Virus, but later EDR had to be added because the preventive solutions were not good enough. However, buying an EDR solution isn’t a silver bullet for companies. Many organizations did not have, and still do not have, the people and knowledge to use these tools properly, he says.
To help organizations with knowledge and expertise, you can secure the help and support of various other companies. Think of MSSPs with a SOC, but also of the solution providers themselves, who can provide a lot of knowledge and expertise. But that still doesn’t get you anywhere, Huikeshoven points out. All the tooling you have running produces an enormous mountain of alerts. Many of them are false positives. So you spend a lot of time filtering the alerts and determining which ones are useful and which are not.
In addition to being time-consuming and costly, false positives can also just be very irritating. Huikeshoven takes the Chrome browser as an example. Sometimes a false positive causes it to be unable to update itself. Google updates the Chrome browser by injecting new code into the existing code. This is also a method that malware regularly employs. The ironic thing about this example is that many updates to the browser are security updates. Your security solution then blocks security updates, which cannot possibly be the point of any security exercise.
Deep learning
In recent years, we’ve seen the emergence of security vendors deploying AI to fight malware. The idea is that this can be used a lot more proactively, so more towards prevention than detection. In other words, it should markedly limit the large number of alerts you get from EDR solutions. When you deploy AI, you generally don’t work with things like signatures anymore, which many traditional security products work with. You train your model in such a way that it autonomously detects suspicious situations and takes action.
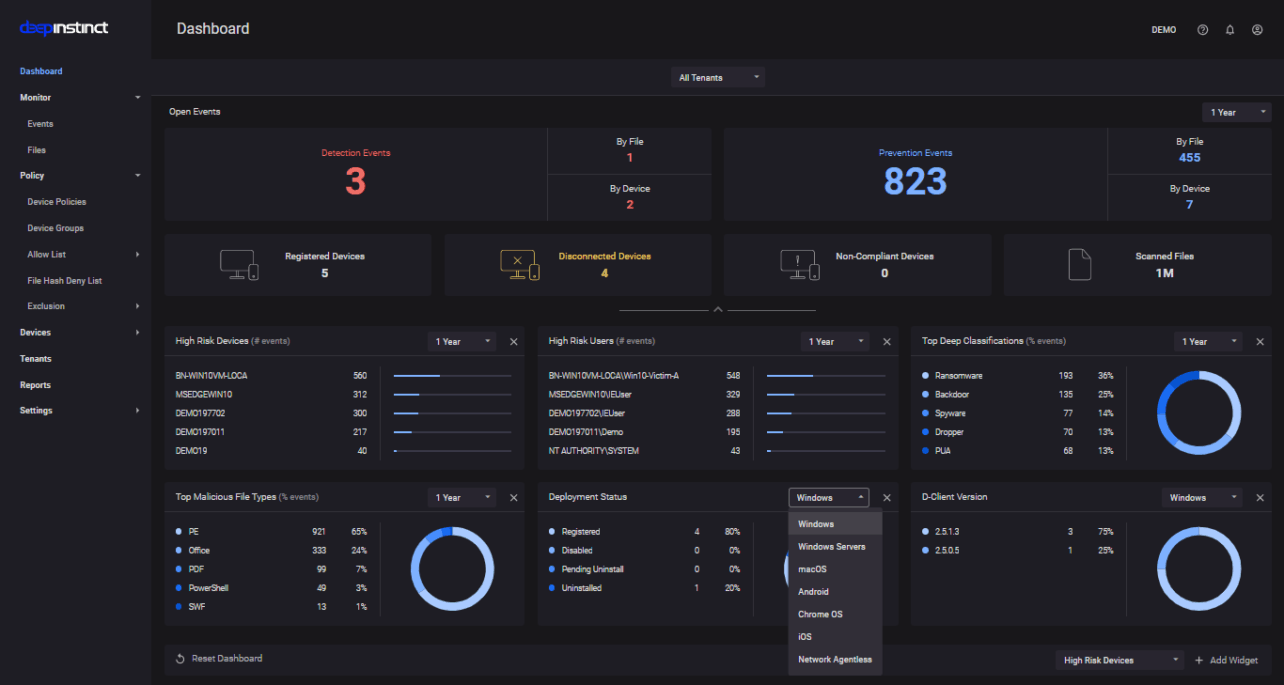
When it comes to AI, cybersecurity solutions have been mostly using Machine Learning (ML) until now, Huikeshoven indicates. ML models usually have to be trained quite specifically and therefore often use customer data. They then always involve humans doing feature extraction on the data they have seen. They draw conclusions from specific behavior of certain software, for example.
In principle, these ML-based tools work fine, Huikeshoven says. That is, if you have the right people to work with them. The problem of the overload of alerts remains. The same goes for the percentage of false positives, he indicates. There are still too many of them.
At Deep Instinct they have chosen not to go the ML route, but to go for Deep Learning (DL). DL uses a neural network that functions like a human brain. You don’t need to train that with customer data. It does need to be trained, of course, but that can be done on generic data. Deep Instinct uses a data lake containing existing malware, self-written malware, but also benign data. No humans are involved. According to Huikeshoven, Deep Instinct is the only supplier on the security market that uses DL.
In addition to static analysis using deep learning, Deep Instinct of course offers much more in the area of endpoint security. Think of the “usual” protection against PowerShell attacks, credential dumping or ransomware, to name a few. Since that’s not the distinguishing part, we’ll only talk about the DL part in the remainder of this article.
D-Brain is fast
As to Deep Instinct’s platform, it is good to know that the neural network runs at Deep Instinct in the data centers. There it is trained using Nvidia GPU-based systems. Twice a year, the neural network produces the so-called D-Brain. That, along with an agent, is what you as a customer install on your endpoints. Deep Instinct supports all common operating systems: Windows, MacOS, Linux, iOS, Android, but also ChromeOS (Chromebooks). The load on an endpoint is also minimal, Huikeshoven says: less than 1 percent CPU load and about 150 MB of disk space.
When we ask Huikeshoven why a D-Brain only comes rolling out of the neural network twice a year, he says that it is simply not necessary to do it more often. It’s not like with signatures, which need to be refreshed very often, or with Machine Learning, where new features need to be defined. The D-Brain is a prediction model, not a static environment that you hold activities against. You don’t need to refresh such a model very often. In addition, it is no easy task to train the neural network, so an update every Tuesday is also just not possible. The agent on the endpoint does get much more frequent updates, of course.
If you have a D-Brain on your clients, that’s basically all you need, Huikeshoven says. You can leave it to function at its own devices. A D-Brain functions completely autonomously. That means it also works offline. Apart from being autonomous, the defining characteristic of a D-Brain is speed. It acts very quickly. Deep Instinct promises that it tells you within 20 milliseconds whether something is malware or not. Partly, this is because it doesn’t have to check anything using signatures, for example. In addition, a D-Brain also does not need to do a hash lookup. In general, you are too late for prevention if you have to do a hash lookup, Huikeshoven argues. In practice this means that a PDF (attached to an e-mail, for example) has already been checked before Adobe Reader has been opened, he indicates.
Other suppliers in the market simply cannot act as quickly, Huikeshoven says. They often link an EDR solution to antivirus, but that means it needs more time. If those tools have to ‘consult’ with each other, you’re already too late.
Does the DL approach of Deep Instinct then replace both your antivirus and your EDR? You shouldn’t see it that way, Huikeshoven points out. EDR remains important in a layered security approach. However, with the deployment of DL you remove all the noise from that EDR solution, so that it also functions better. In other words, you’re being much more proactive. Basically, things like EDR and the concept of rollback are reactive and therefore too late.
Warranty on false positives and against ransomware
Speed isn’t the only distinguishing feature of the Deep Instinct approach, says Huikeshoven. Deep Instinct also outperforms the competition when it comes to false positives, he says. They even dare to put a warranty on it. If a customer receives more than 0.1 percent false positives, they will be compensated (up to a certain amount). This hasn’t been legally approved in all regions, so you won’t be able to use it everywhere yet.
Getting as few false positives as possible is very important, as we have already indicated above. A false positive does not only cause certain applications to suddenly stop working, it also results in a lot of extra work. Work you have to spend resources on for nothing. This makes the warranty that Deep Instinct gives on this point quite interesting. Assuming they can live up to it, this is unique in the market as far as we know. Other providers of endpoint security based on AI also claim to produce very few false positives, but don’t make any promises or give out warranties.
Deep Instinct doesn’t limit its warranties to false positives, however. It also gives one on ransomware. If you use Deep Instinct and you become a victim of ransomware, then Deep Instinct will pay the costs of recovering from that attack, up to a maximum of $3 million. Again, this warranty isn’t available yet in all regions, but will be in the near future. Even though a warranty like this one will undoubtedly be accompanied by some fine print, it shows that Deep Instinct has a lot of confidence in the product.
Current restrictions of the platform
Deep Instinct supports many different platforms, as we have already seen. However, there are limits to what it can currently protect. This is to be expected, because at the moment it depends on the installation of an agent. If this is not possible, then Deep Instinct cannot protect it on the endpoint itself. An example of such an endpoint are IoT sensors. These usually run in the firmware and offer no possibility to install anything.
Of course, you can solve such a limitation by providing protection as close as possible to such an endpoint. However, this is not ideal, especially if, like Deep Instinct, you place the emphasis on prevention. If malware can get in via an endpoint, you’re already too late for that. When we asked Huikeshoven how they were going to tackle this, he said that they would be coming up with a solution for this in the foreseeable future. In other words, there will also be an agentless option, for what Huikeshoven calls beyond perimeter security. As an example, he mentions sending a file to or from cloud or local storage. In such a scenario the file goes through the D-Brain before they actually go anywhere. A similar approach should also work for IoT devices.
In conclusion: how do deploy it and what does it cost?
Now that we have a clear picture of what Deep Instinct promises and how the product is put together in general, we still have some practical questions. For that we talk to Joost van der Spek, the Regional Sales Manager of Deep Instinct for our region. First of all, the question of how an organization can purchase the offerings of Deep Instinct. According to Van der Spek, this can be done in two ways, depending on how big the organization is. The dividing line is at 1000 endpoints. Below that it goes through an MSSP, above that Deep Instinct offers the choice between a direct subscription model or through an MSSP.
Furthermore, there is a distinction in the support that Deep Instinct offers. Here too, it offers two flavors: standard and premium. Mind you, Van der Spek adds, the product is the same for both support levels, it’s really only about the support. With premium you get 24/7 support and extra warranties.
Then the costs: these are always a bit tricky to determine. The final price depends on all kinds of factors, especially if MSSPs can partly determine them. So we can only give the MSRP: that is set at $47 per endpoint per year with standard support and at $57 per endpoint per year with premium support. The subscriptions also include a one-time so-called Enterprise Quickstart Fee.
Explainable AI and the D-Brain
We have noticed a trend in recent years towards explainable AI. Do they get many questions about that at Deep Instinct? An autonomously operating product of a neural network can be seen as a black box, whose actions are therefore far from explainable and transparent.
When it comes to explainable AI, we probably need to differentiate between different market segments. At Deep Instinct, they don’t get any questions about this. This makes sense, because the AI doesn’t necessarily need to be explained. This is different in customer engagement applications for example, where an AI needs to decide on next-best actions for customers environment at, say, an insurer. There you need to be able to explain why an AI makes a certain suggestion to a customer. In cybersecurity, you focus purely on the outcome. If prevention is possible again thanks to a neural network, then it doesn’t matter so much whether you know exactly how this works.