Researchers have designed an AI framework that recommends cities and regions lockdown and home isolation strategies to combat the spread of the coronavirus. They claim that their AI is superior to the modeling tools used so far.
Artificial intelligence, according to researchers at Microsoft, the Indian Institute of Technology and TCS Research, would automatically learn and take into account complex issues. These include infectivity, gestation time, duration of symptoms, chance of death, population density and movement tendency.
The researchers published their findings in a paper. If the peer review process supports the researchers’ claims, the framework could be useful for organisations and governments in countries struggling with the spread of the corona virus.
Structure of the AI framework
The framework uses a model that links points (nodes) to each other, as shown in the picture below. Each node represents a city or region, where the size of a point shows the population of an area. For example, areas that have few inhabitants and are far away from large areas will not easily be connected to such a large area.
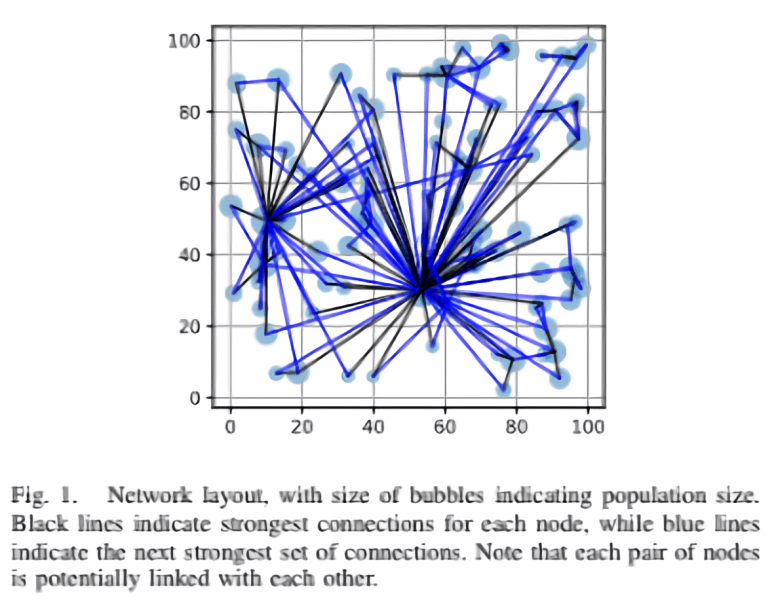
The researchers then modelled the best disease parameters currently available for the corona virus. They assumed an incubation period of 5 to 10 days, an infected period of 7 to 14 days, an 80 percent chance of visible symptoms, a mortality rate of 2 percent and a 100 percent chance of transmitting the virus if a susceptible person comes into contact with an infected person.
The researchers assumed that people could travel to and from other areas. People with visible symptoms of the coronavirus were not allowed to travel to other nodes in the model, but asymptomatic people could. They also took into account the fact that although symptomatic people were quarantined within one node, some people did not adhere to the quarantine and moved within that node.
The researchers also drew up a number of simple policies for lockdowns, assuming that each node would be able to open or close once a week. They then defined a set of policies that ensured that areas went into lockdown when they exceeded a predetermined threshold of 5 percent, 10 percent, 20 percent, or more than 100 percent.
Reinforcement learning
Finally, the team trained a Deep Q Network for reinforcement learning (an algorithm that stimulates models if they do a good job) which requires the models to make an ‘open’ or ‘lockdown’ decision on a weekly basis by node by performing simulations on the spread of the corona virus. The algorithm identified the optimal policy for lockdowns by means of values. For example, the calculation methods assigned 1 point for a lockdown day or an infected person, whereas a deceased person stands for 2.5 points.
The research team ran 75 simulations, each lasting 52 weeks (364 days). In this way, they were able to determine that even with limited measures, areas had fewer infections. The measures take the fact into account that infections increase within an area, and that the size of the population contributes to this. Larger areas were closed as soon as the infection started to spread, in order to smoothen the spread to other areas.
The researchers stressed that they are not experts on infective diseases, and that the AI model does not take geography into account. Also, no real data were used for the simulations.