Databricks is convinced that the lakehouse will help them kick-start a new industry trend. After data warehouses advanced the business intelligence (BI) world and data lakes simplified data science, the next step should be a data pool to support all kinds of applications. Are we entering a new era, or is it only a marketing buzzword?
Databricks builds new frameworks and paradigms to simplify data. An example of this is Apache Spark, the analytics engine that simplifies and accelerates the traditional extract, transform, load (ETL) process. To accelerate the development and adoption of Spark, the company chose an open-source approach and donated the software to The Apache Software Foundation.
The lakehouse idea should follow the same path. During the virtual Spark + AI Summit, Databricks explained what lakehouses do and how they will improve.
Lakehouse as a foundation for all data use cases
If a company uses multiple systems, databases and applications, there are many different types of data. Data that is often written in many different ways. To analyse this data, you will need to combine all of the data in one central location. It’s important that this location allows the data to work together and creates a logical structure. Traditionally, data warehouse technology is often used for this purpose, while data lakes are also widely used. They each have their advantages and disadvantages.
For example, a data warehouse collects sales data from 40 countries. It includes historical data, as well as current sales figures. This information will be prepared for analytics, in most cases BI, to understand what it says. Analysts will be able to spot trends at a detailed level, and it is possible to predict what will most likely happen in the future. A data warehouse, therefore, enhances responding to potential opportunities and threats to the business, while also enabling cost optimisations.
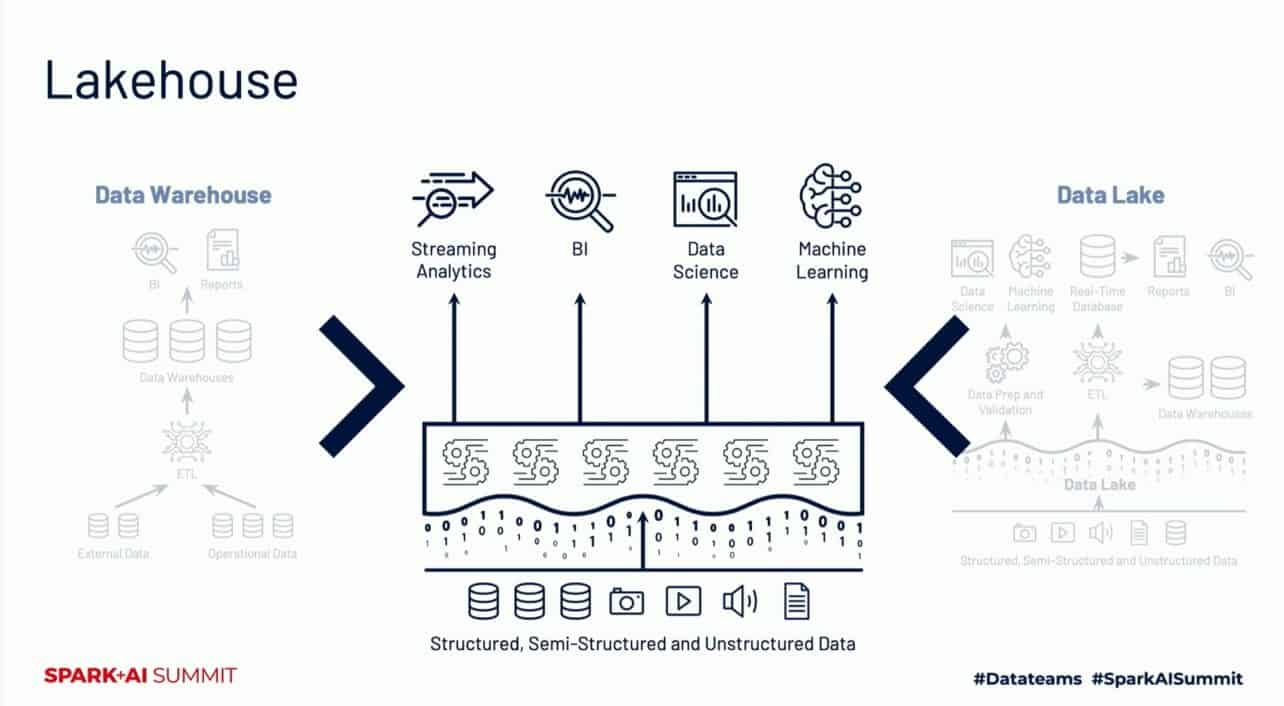
Data warehouses, however, lack support for video, audio and text data. This data is being generated and used more and more for analytical purposes, such as machine learning and data science. To properly execute this type of analytics, data lakes are often used. A scalable technology that supports structured data, unstructured data and semi-structured data. For example, data from databases, emails and video streams. According to Databricks, data lakes are very suitable for data science and machine learning, and often exists along with data warehouses. After all, data lakes offer limited BI support and are more complex than data warehouses.
That is why Databricks, with its lakehouse vision, combines the technologies. The lakehouse promises the structured, BI and reporting advantages of data warehouses together with the data science, machine learning and artificial intelligence (AI) benefits of data lakes.
What does a lakehouse look like?
The lakehouse looks at some parts like a data lake, as it handles more or less the same types of data. A structured transactional layer subsequently delivers quality, structure, governance and performance to the data. Databricks uses the Delta Lake project as this layer, which is designed to tackle some traditional problems. Obstacles that Databricks encounters are, for example, incorrect reading tasks within the data lake, performing real-time operations being hard, modifying data being hard.
One of Delta Lake’s tasks is to save events in a log. It allows a layer to monitor the actions within the data lake. Actions are only approved if they are fully executed. Otherwise, the action is erased and might need to happen at a later time. It guarantees data quality: the data is complete and up-to-date, changes and modifications are fully implemented.
Further quality should be assured through schema validation. These schemas, often a snowflake or star schema, are used to organize data efficiently. A check by Delta Lake should ensure that data adheres 100 per cent to the applied schema. Any inconsistencies are stored separately so that users can decide what to do with the data.
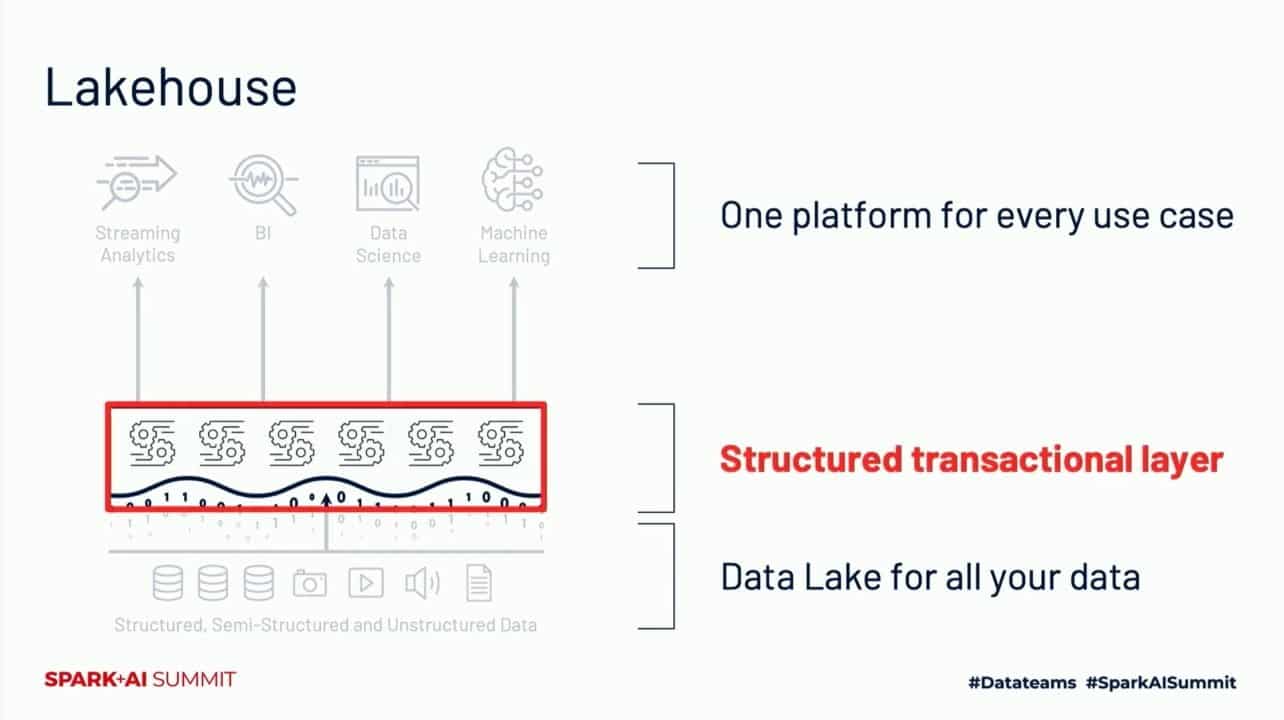
Databricks also uses indexing technology to improve the performance and structure of data lakes. According to the company, a traditional data lake is often a combination of millions of small files with a few huge files. By automatically optimizing the layout, for example, with a layout that can handle frequently used queries, users gain quick access to the data.
Finally, Spark is used to simplify the setting up of data pipelines and improve efficiency. Spark is built to process large amounts of data. Using Spark should make it easier to handle large amounts of metadata in the data lake. According to Databricks, companies often encounter problems when they want to use large amounts of metadata in traditional data lakes.
Confidence in project should stimulate adoption
Databricks is convinced the best of data warehouses and the data lakes should converge to realise more simplicity, benefits and applications. As many businesses as possible should be able to benefit. That is why Delta Lake is part of The Linux Foundation, to accelerate its development.
Visualisation service Redash has also been acquired by Databricks. This technology helps users to understand queries by visualising the query. A dashboard contains charts and other kinds of visualisations, which makes the query easier to understand. This way, not only does the data scientist understand what the data says, but C-level also understands the data. It enables the entire organisation to make decisions based on data.
In the end, Databricks is committed to ensure lakehouses will succeed. The goal of advancing the AI and data world is also clear, although it will take some time to realise a large-scale adoption. Companies often rely on great products for data warehousing, among other things, which raises the question of whether they want to invest in a lakehouse. The lakehouse vision needs time to prove whether it lives up to its potential.
Tip: Databricks sees open-source as the way to innovate in data science