Despite all the hype, AI has a problem. As compelling as the skills of ChatGPT or a self-driving car may already be, they don’t simply learn from their mistakes. At MIT, researchers discovered “liquid neural networks,” which could solve many existing challenges for AI. What does this innovation entail? And what problems could it solve where AI has so far fallen short?
A research team at the famed MIT saw what problems AI models are facing, long before ChatGPT took the world by storm. To ensure an accurate output, gigantic data sets are an essential component. On top of that, that information must be thoroughly checked for inaccuracies and be balanced to remain free from unwanted biases. On top of that, training and running these AI models takes an enormous amount of energy, money and hardware.
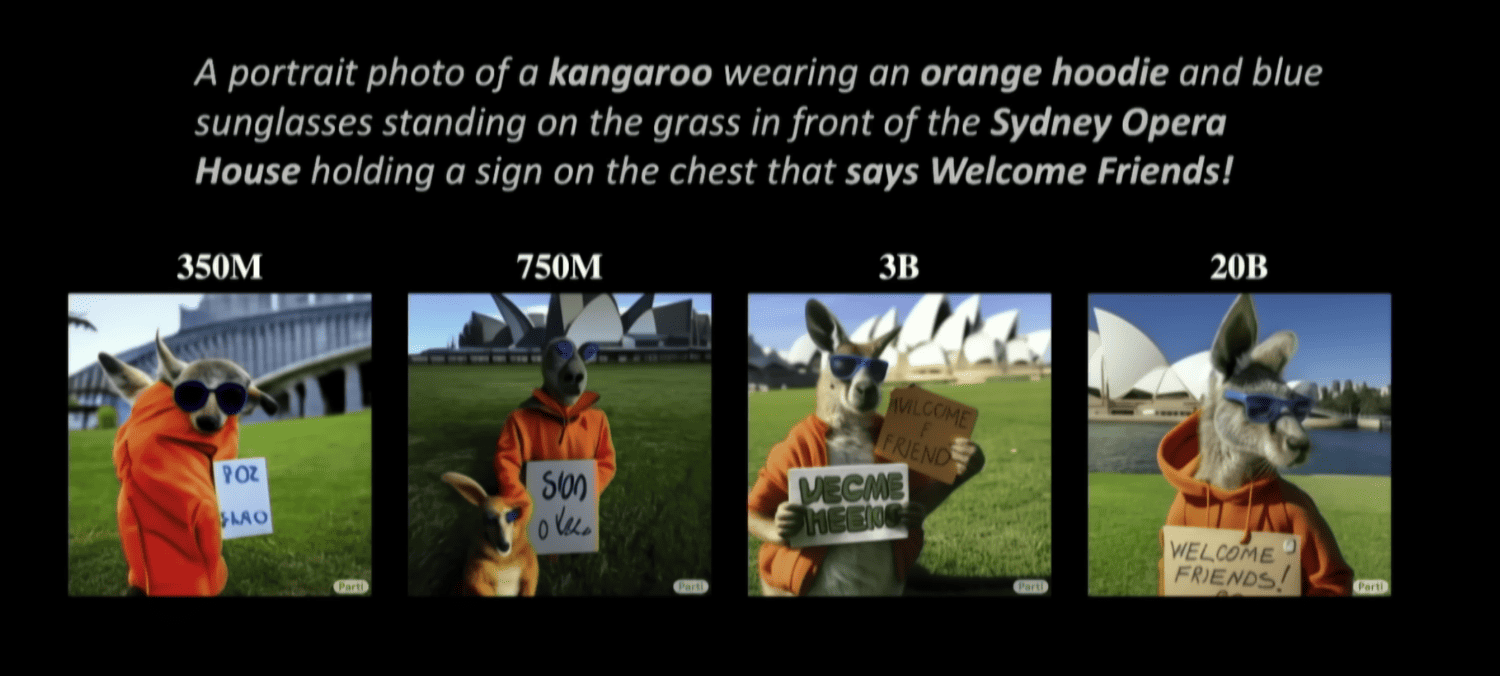
The result is sometimes commendable: many outputs from chatbots appear quite human, which in itself is quite an achievement. It is merely one example of AI being used in the wild, but it has been in vogue this year as a showcase of the technology. Generative AI, however, remains mostly a black box, especially since OpenAI does not reveal much about how its most advanced model (GPT-4) is put together. Despite all the computational firepower, these AI models hallucinate facts with the same confidence as they would espouse truthful statements. An example of how things could be different was Dolly 2.0 from Databricks, which showed impressive results achievable with a small AI model and very specific data.
Back to basics
There are more AI models making the rounds than just large language models, but they all seem to suffer from similar problems. A deep learning supersampling model from Nvidia, for example, can smartly upgrade a 1080p image to 4K like no other with convincing results, but it, too, has no true notion of what it is doing in the slightest. In addition, AI models are static until they are retrained or parameters are tinkered with. They rely heavily on labels and refinement of datasets, while outside the context of a test scenario, they usually miss the mark.
At MIT, a 2020 research team went back to basics: the inspiration for artificial intelligence starts with the real world, i.e., natural intelligence. Speaking to Quanta Magazine, Ramin Hasani and Mathias Lechner revealed that they drew inspiration from a roundworm about a millimeter long, the Caenorhabditis elegans. It is one of the few organisms with a fully explored nervous system, it is able to move, eat, reproduce and most importantly, learn. They seem to cope well with changing environments in nature. This is exactly where liquid neural networks, which borrow their design from this roundworm, can leapfrog AI development.
The test scenario
The MIT researchers have been making their findings known for some time throughout 2023, but their examples and explanations remain mostly the same. They developed an AI model in which the number of neurons still remains very manageable and easy to oversee. In his test scenario, Hasani talks about 19 neurons that can adapt to the output they help produce, providing feedback on-the-fly on their own actions. The team tested liquid neural networks against traditional AI models in two scenarios: driving a car on a closed test track and steering a drone toward various coloured objects. The kicker: they did not tell the model what the task was.
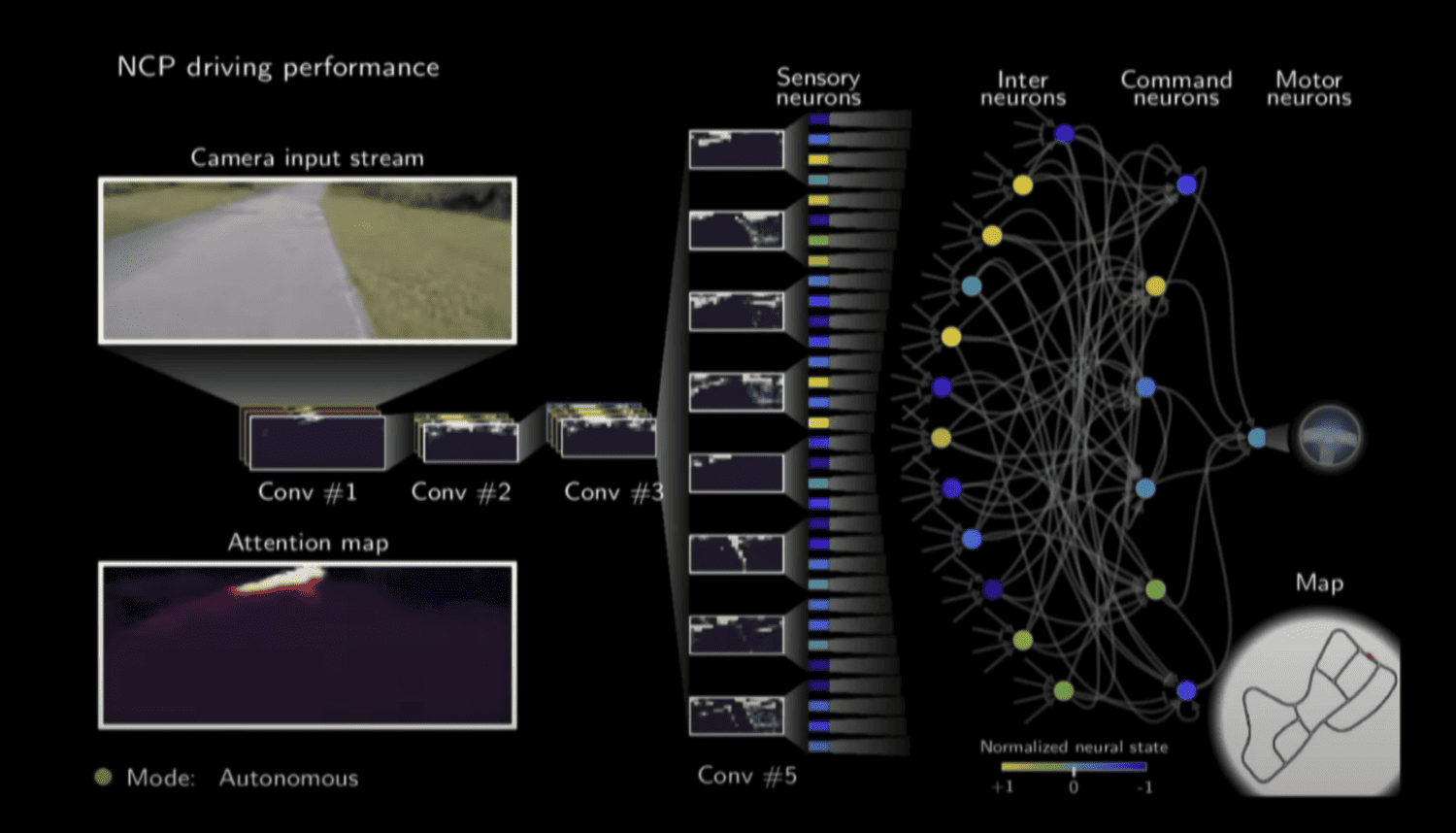
In the tests, it was noticeable that ordinary AI models cannot distinguish between primary and secondary issues without additional help. In the driving test, the camera heatmap showed that conventional AI may focus on the grass along the road or points of light among the trees. It will shift that focus on occasion, but in a haphazard way purely based on the visual feedback we get to see. The liquid neural network, however, “looks” the way a human does: it focuses on a distant point on its trajectory and keeps an eye on the edges of the road as it does so. In addition, the visual representation shows that the MIT model remains neatly stable with this observation.
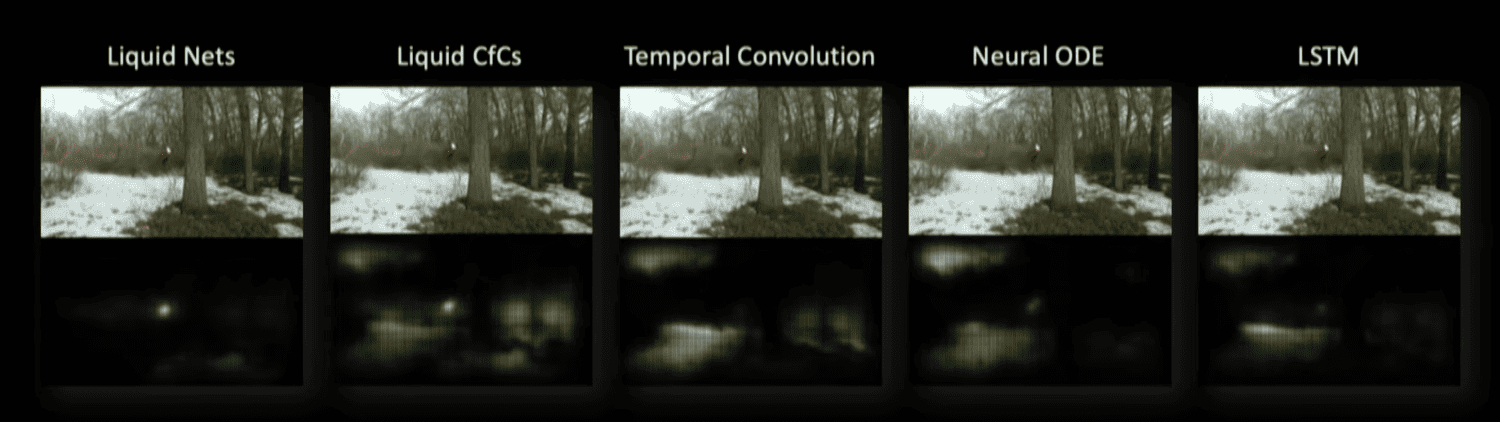
Promising but early
Liquid neural networks are a lot more compact than, say, an LLM like GPT-4 or LLaMA 2. It is inconceivable for a robot, self-driving car or delivery drone to be able to run such large models internally: it requires a whole fleet of robust hardware. Future hardware gains aside, you can’t fit an AI-capable server in a portable piece of kit.
Daniela Rus, director of MIT CSAIL, told VentureBeat that the inspiration behind liquid neural networks came in part from wanting to put AI to practical use. The compactness of liquid neural networks are promising in that regard.
While companies are currently buying every Nvidia chip that comes near them, the AI hype seems to have taken a direction. However, even generative AI is still in its infancy and the growing pains are palpable. An alternative like MIT’s is therefore promising, but even there it will be a case of wait and see.
An important feat for it, though, is that the over-sightedness of individual neurons will make it a lot easier to find the reasoning behind the output. Since the AI Act from the EU can bring the heat to to big tech, it is a good time to look around at how AI can be manageable, reliable and workable. Liquid neural networks are an intriguing phenomenon in that regard. However, it will be some time before it fuels any next hype cycle.
Also read: AI Act: legislation that plays catch-up with a new reality