VAST Data is expanding its Data Platform with the addition of InsightEngine, in partnership with Nvidia. With this, the company aims to offer organizations that deploy Retrieval-Augmented Generation (RAG) for their (inferencing) workloads more scalability and less complexity. It is the latest move by the company that aims to build and be the best data infrastructure for AI workloads.
VAST Data has been out of so-called stealth mode since 2019 and has been pushing hard in recent years. There have already been the necessary rounds of investment and the company has also launched a real (online) event with Cosmos today. Today, together with Nvidia, it is also announcing the VAST InsightEngine. You can see that as a culmination of the company’s vision so far. To some extent, it is also a logical step. Last year, the VAST Data Platform came out. This year saw the preview of VAST DataEngine, the runtime of VAST Data. VAST InsightEngine uses the VAST DataEngine and is the first dedicated workflow running on the VAST Data Platform.
We can imagine the above paragraph has left you somewhat puzzled by the terminology. Hence, we will first take a step back and introduce the various components below. After that, dive into InsightEngine. If you don’t want to get the brief breakdown of VAST Data’s offering, you can also scroll down a bit and continue reading under the heading VAST InsightEngine.
The basics: VAST DASE
Before we talk about VAST DataEngine and VAST Data Platform, let’s take a quick look at VAST Data’s foundation. VAST Data is a relatively young company in the market in which it operates. Previously, we would call this the storage market. However, that name no longer does justice to what this type of player does. Of course there are appliances where the data is stored. However, it is much more about the platform on top of it. That platform needs a foundation. That foundation goes by the name of DASE.
DASE stands for Disaggregated, Shared-Everything. Compute and storage are decoupled from each other, which greatly benefits the scalability of the infrastructure. If you need more storage, you only need to add more storage, not more compute right away. In addition, it is a highly simplified architecture, which also does not involve tiering. All the traditional tiers (hot, cold, archive) are in the same (all-flash) tier. This is possible because everything can basically communicate with each other within this architecture. That’s what the Shared-Everything part in DASE stands for. Finally, communication between the different parts is also lightning fast thanks to NVMe. That doesn’t change when companies need to scale up or out.
The above description of DASE is, of course, somewhat brief. For a more comprehensive version, please refer to this page from VAST Data itself.
VAST Data was there early
With DASE, VAST Data did in 2019 (actually before, because the company was in stealth before that) what many older players in this market did or do much later. Just last week, for example, we attended NetApp Insight. That company announced a new, disaggregated architecture during the event. That company (obviously) also recognized the need for this. However, for a 30+-year old company it takes some time to get this right. We understand that it is already running in NetApp’s labs. However, it is not clear when the company rolls this out to its customers.
VAST Data did not have any legacy when it developed DASE. That gave and gives the company a big edge in terms of modern disaggregated architecture. When it comes to the depth of its offerings, for example in terms of features, the company still needs to make the necessary steps to get up to the level of the older players. Or maybe not. VAST Data is primarily targeting new AI-driven workloads of organizations with its own architecture. It wants to develop a data platform for that. That also automatically means that right now not everyone is a customer for VAST Data. Or not yet, assuming everyone will eventually jump on the AI train.
VAST DataStore, DataBase, Data Platform and DataEngine
Now that we have the fundamental architecture somewhat in focus, we can look at the other terms you often encounter within the world of VAST Data. On the one hand, there is the data part, which consists of the DataStore and the DataBase. The VAST DataStore is the company’s file and object store (or structured and unstructured data). For the VAST DataBase, VAST has done another consolidation, which could be likened to folding together the various tiers above. That is, VAST has managed to build a database that also immediately includes a transactional data warehouse.
Furthermore, it is also interesting that the VAST DataBase can also handle vector data. That is the data that AI applications make use of. VAST Data can convert unstructured data into structured data and write that into the VAST DataBase. After this is done, queries can be fired on this data. Since there can be a lot of value in unstructured data, including for AI applications, this makes much more data available to extract value from. Converting and making unstructured data queryable makes sense today because neural networks now understand this type of data very well, Jeff Denworth, co-founder of VAST Data indicated during a briefing we attended.
Both the VAST DataStore and the VAST DataBase are part of the VAST Data Platform. That platform ensures that these components integrate optimally with each other, is the idea. To do that, it needs the necessary intelligence. That comes in the form of the VAST DataEngine. Until today, this is the most recent addition to VAST Data’s offering and is a collection of serverless functions and triggers. These should make the files, objects and tables come to life and start adding value to the user.
The VAST DataEngine lives in a container and can be deployed on CPUs, GPUs and DPUs. It ensures that the logic needed to maximize the value of the DataBase and DataStore is part of the Data Platform. Finally, to tie things together a little better at scale and in distributed environments, there is the VAST DataSpace. This is a global namespace that ensures that all data is always accessible everywhere and is optimally performing and secure.
VAST InsightEngine
Above, we explained in a nutshell what VAST Data has to offer. You could call that the basics. Today, with VAST InsightEngine, the company is announcing the first so-called application workflow running on the VAST Data Platform. This is a workflow focused on a specific task. This specific task is to ingest, process and retrieve all enterprise data in real time.
To make this possible, VAST Data has a native integration between its own Data Platform and Nvidia NIM microservices to immediately provide the (unstructured) data coming in with the necessary semantic values. To do so, it uses the muscle that Nvidia can provide in this collaboration. InsightEngine uses VAST DataEngine to send a trigger to the Nvidia NIM embedding agent the moment new data is written to VAST’s systems. This ensures that unstructured data is converted to vectors or graphs in real time. This data is then almost immediately available and searchable for AI tasks.
VAST InsightEngine wants to enable companies to use real-time Retrieval-Augmented Generation (RAG). With RAG, you always have external data sources that you leverage to arrive at insights. If those are unstructured data sources, you need to convert them first. That is now possible, all within VAST Data’s platform. So here again, we see the pattern that VAST Data mainly wants to reduce the complexity of processes and workflows. That results in a platform that is a lot less complex than it was until now. Indeed, it automates the workflow around data pipelines for AI applications. It is also a lot faster without sacrificing data consistency and security, according to VAST Data. With AI in mind, this is definitely nice. After all, that already adds enough complexity for organizations.
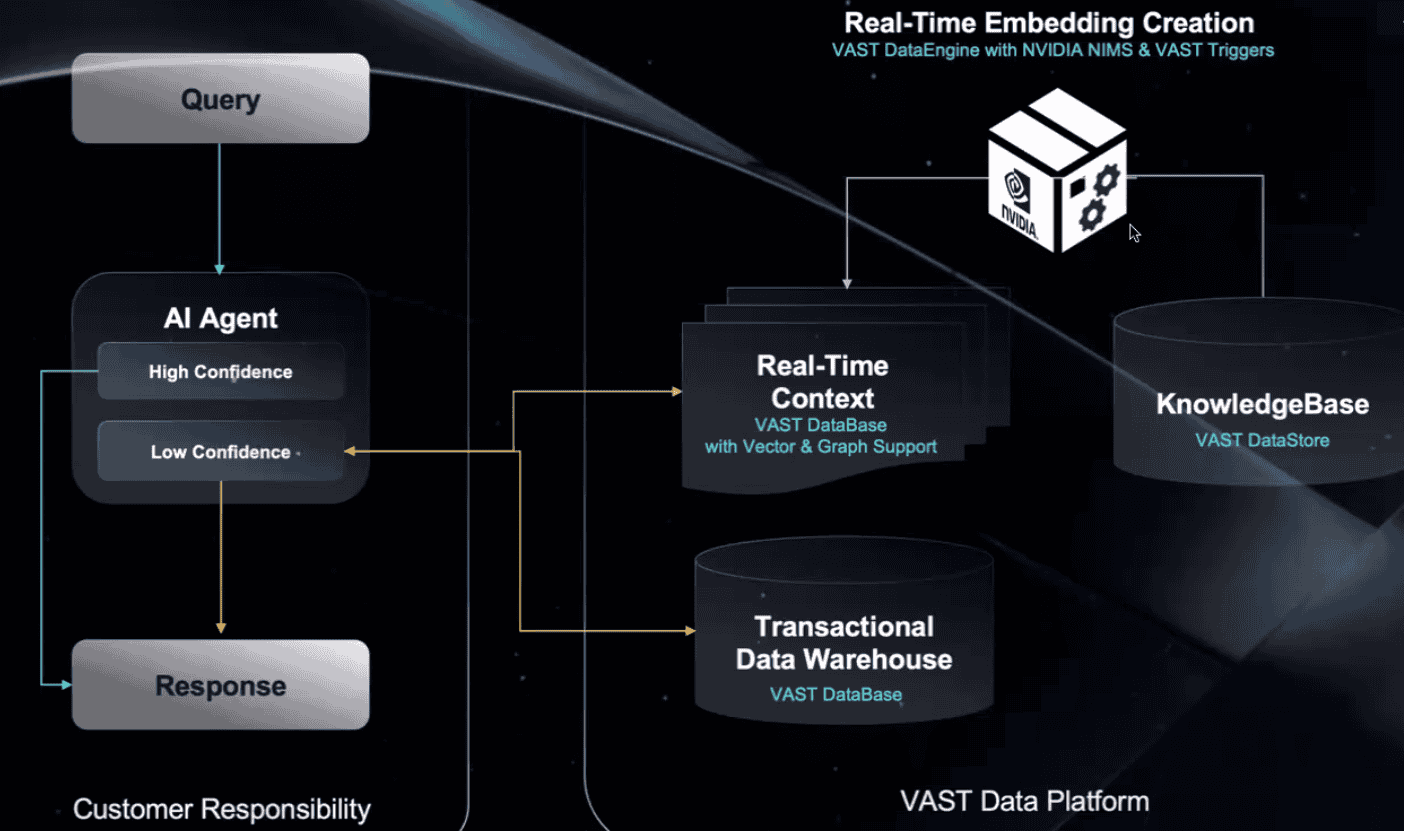