One of the key issues for utilizing generative AI is how to keep data secure. As a company, how do you ensure that you can benefit from the nascent technology without putting your proprietary data at risk? VMware and Nvidia have partnered up to make this a possibility, and are unveiling what they’ve called the Private AI Foundation. VMware is also working with several AI-related companies to encourage an open ecosystem for the technology.
VMware and Nvidia are no strangers to one another, as their collaboration has now been going on for a decade. Together they have innovated in various fields, from graphics simulation techniques, HPC solutions and training methods for machine learning. Now, they’re developing a solution in gen-AI, something the entire tech industry is familiar with by now. Yet despite all the developments in this field, there is still an unanswered question: as a company, how do you safely deploy your data while realizing the potential of AI?
The utility of AI is clear, but how do you apply it carefully?
A distinctive aspect of generative AI is that its specific application can vary enormously from company to company. Financial institutions can use it to detect fraud, but it can also simulate all kinds of systems at wide-ranging scales, from molecular structures to entire factories. Perhaps the best-known application is the deployment of AI assistants that can generate programming code, insights about customer behaviour or convincingly humanlike text. VP of Enterprise Computing at Nvidia Justin Boitano cites research from Accenture that indicates how promising AI is for businesses. Those researchers found that a large company is 2.6 times more likely to achieve a 10 percent increase in revenue if they deploy AI effectively. Boitano talks about a tenfold increase in our productivity that could theoretically benefit any business. But there, of course, lies the crux: how do you do that?
Vice President of Product Management vSphere at VMware Paul Turner names the most valuable asset that companies have in their hands: their own data. If all goes well, organizations have their own data secured and centrally collected. Generative AI can aggregate this information and transform it into useful insights, such as in the examples mentioned earlier. The technology sometimes sounds woolly, but Turner sums up its usefulness to his own employer as follows, “Within VMware, we take our code capabilities, our APIs and all of our interfaces to generate code samples for you. If you want to migrate a VM within your environment, we can automatically create the code sample for that. In addition, we bring together our knowledge base based on 25 years of knowledge base articles to generate automated answers for customer support. All companies can do something like that.”
The choice for businesses: public or private
Within the public cloud, there are plenty of advantages to be named. The scalability of a remote datacenter and 24/7 availability are very enticing. Also, it is unimaginable to run an AI model the size of a GPT-3 or GPT-3.5 on in-house infrastructure, let alone GPT-4. Still, VMware advises against this plan of action. The enterprise data is sent toward a public cloud, with the danger that someone might manage to steal it. Additionally, for many models on the public cloud, it is impossible to determine what data they’ve been trained on, making the output difficult to control or verify.
In short: another solution is needed. You can’t manage it all on your own as an organization, and you don’t want to expose yourself to the dangers of public cloud. Turner mentions Meta’s LLaMA 2 model, which is publicly available. You can take this model on-prem, (for example, the 70B variant, i.e. with 70 billion parameters) feed it with your own enterprise data and arrive at a workable solution. “Put your data into those models and refine them with that. What you’re basically doing is adding the flavour of your data to transform it into something you get real business value from.” Together with Nvidia, Turner promises that VMware can get this done inexpensively and easily.
An off-the-shelf package
Logically, companies often have no idea where to start when it comes to AI. For that reason, VMware and Nvidia are taking a platform approach. They have devised an infrastructure that is as approachable as possible: companies already deploying VMware’s cloud infrastructure can quickly take advantage of it. They also promise to use the best technologies currently available.
From Nvidia, customers can use the Nvidia AI Workbench and NeMo Framework to refine AI models to their liking. VMware’s offering hooks into that: it has developed specific off-the-shelf VM images suitable for deep learning. Essential to this is the Vector Database, which is needed to do AI inferencing (or AI’s predictive capabilities based on existing data).
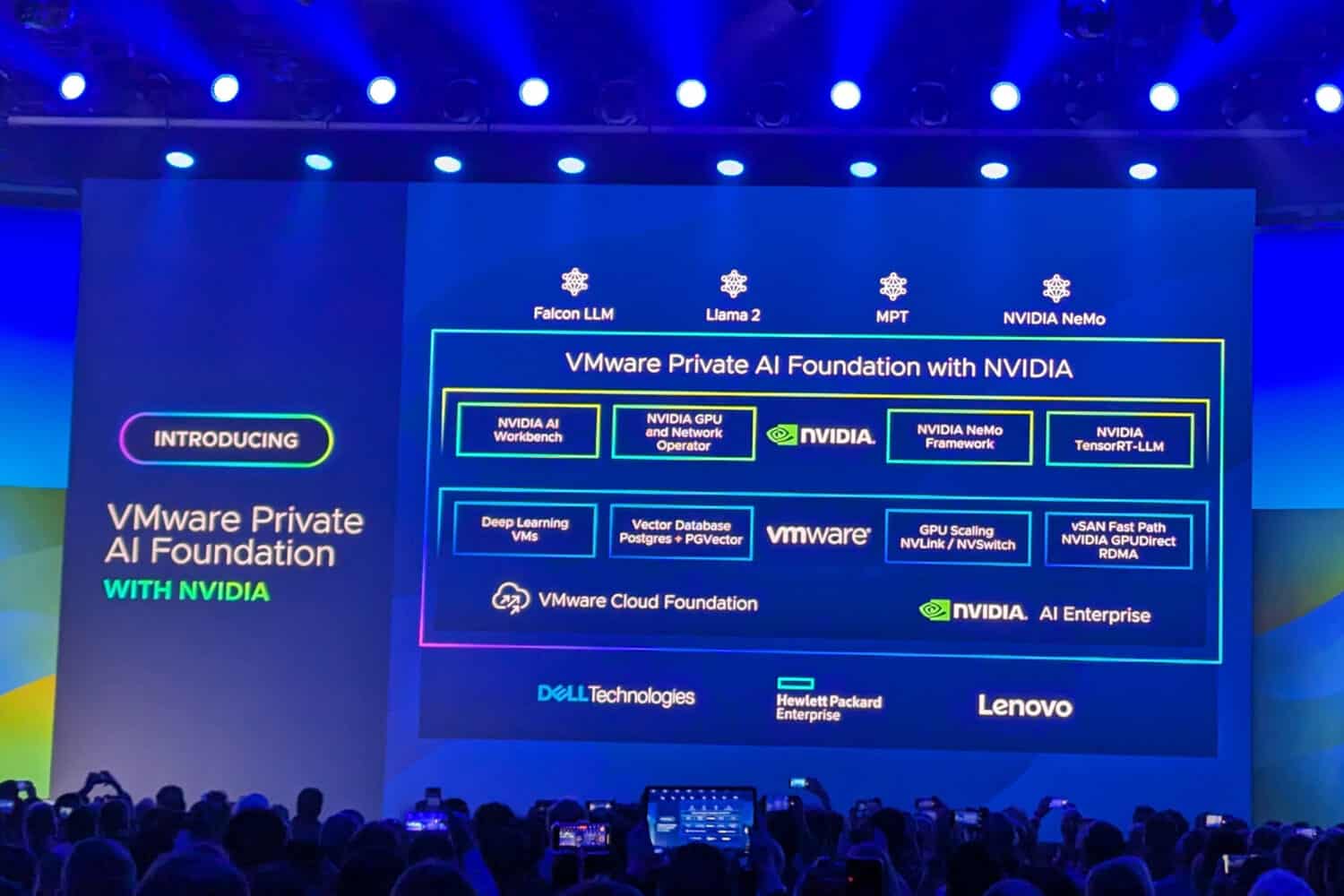
Not every AI workload is the same and not every company needs data at the same speed or models of the same size. That’s why this Private AI solution is scalable, with vSphere VMs capable of deploying up to 16 GPUs. They communicate with each other via the extremely fast NVLink interconnect and can be addressed directly from NVMe storage without needing to talk to the CPU or other components. This is even possible from server to server, minimizing latency. The solution utilizes the Nvidia L40S, a graphics card announced just two weeks ago. Each GPU has 48GB of video memory. To run a model like Meta’s LLaMA 2 in real-time, for example, requires 140GB and thus a minimum of four GPUs working together. There are plenty of alternatives here, ranging from larger to much smaller models, which is why this scalability is very useful.
Delivery through partners, then get to work
Running it all requires more than software from VMware and Nvidia and some impressive GPUs. Datacenter vendors Dell, HPE and Lenovo can build full-stack platforms suitable for generative AI. These are new, optimized systems that can be placed within server racks. It’s also possible as customers to receive just the software and build their own systems.
All this means that, according to Boitano, companies are starting off with 95 percent of the work already done. What remains is the provision of your own data that varies from company to company and to ensure that the LLM you choose can assist you with the desired task. Through the software provided, everything is said to be easily customizable as desired to achieve this incrementally. The end result is a “new model with a nuanced understanding of your own company data while remaining private,” Boitano said. “It allows one-click migration from laptop or PC to the datacenter. That’s where most of the computing of large language models will take place.” Fine-tuning an LLM of 40 billion parameters can be completed by 8 L40S-GPUs within 8 hours. Then, Nvidia’s software ensures that the deployment of these AI models is as efficient as possible.
With the Private AI Foundation, VMware and Nvidia promise a workable, relatively simple solution for running generative AI within corporate walls. Step by step, customers can realize the deployment of AI without additional fears of data breaches or frustrations over uncontrollable models. However, there is still a bit of a wait: Private AI Foundation should appear in early 2024.
An open ecosystem
In addition to Nvidia, VMware has a number of other AI-focused companies to partner with. It summarizes its system of partner solutions and proprietary applications as the Private AI Reference Architecture. For example, within VMware Cloud Foundation, it has applied the Ray “unified compute framework” for optimizing compute footprints. Domino Data Lab also partners with VMware and Nvidia to provide a specialized AI platform for financial institutions. Hugging Face provides a programming code assistant that VMware itself also uses internally; the latter is therefore publishing an example for customers of how this AI tool can be run as quickly as possible within existing VMware infrastructure. Finally, VMware cites Intel: its 4th-generation Xeon processors contain AI accelerators that should be optimally utilized within VMware vSphere/vSAN 8 and Tanzu.
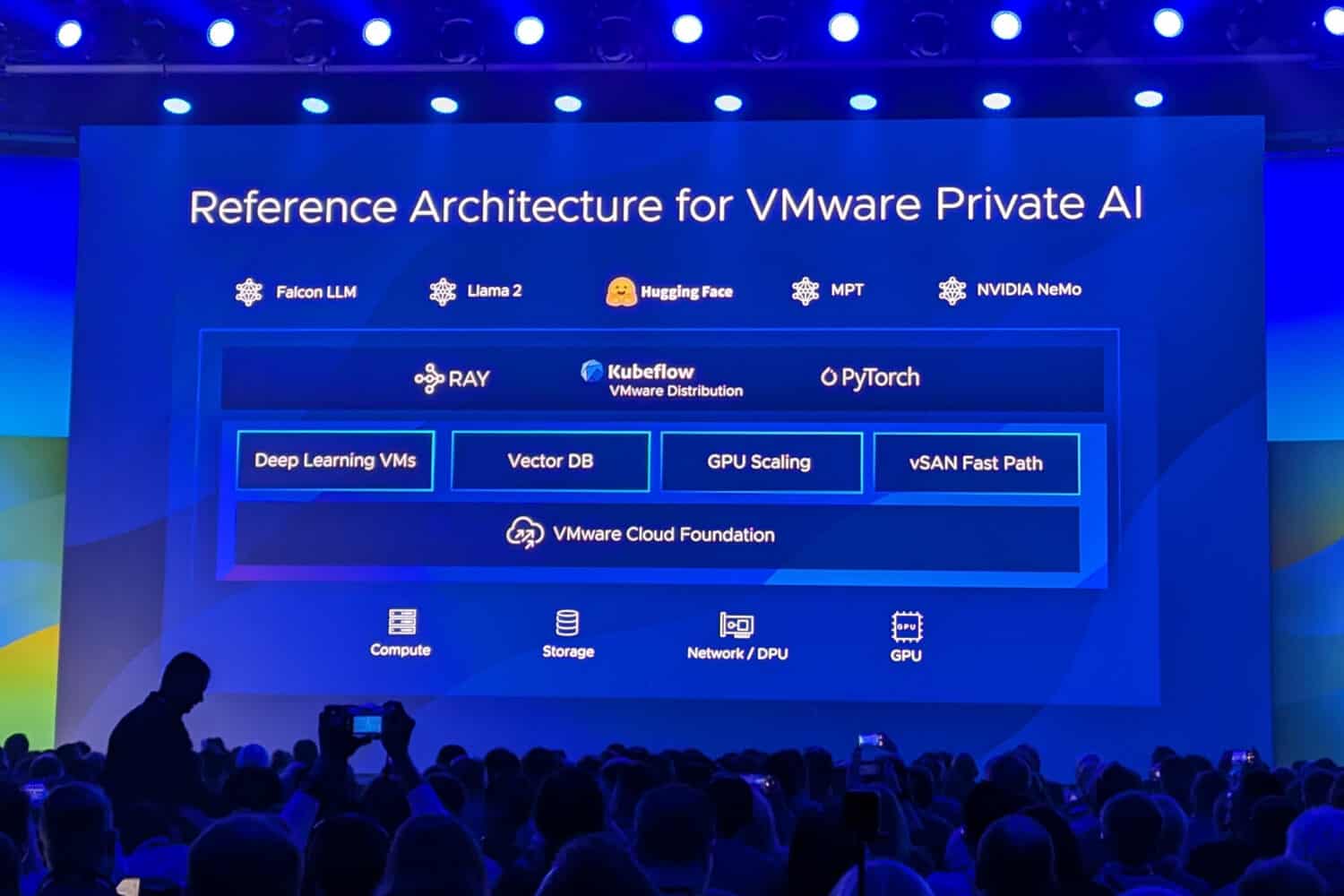
During VMware Explore in Las Vegas, there will be showcases of these innovations over the next few days. We have also already published an overview of the modernization VMware has implemented within its own Cloud Foundation.
Read that article here: VMware Cloud up to date again with NSX+, vSAN Max and better security